Are there variations of the regular runtimes of the Big-O-Notation?For every computable function $f$ does there exist a problem that can be solved at best in $Theta(f(n))$ time?Progressive discrete multifunction optimizationInteger Programming - packing wolves and sheepHow to compute amortized complexity of n runs of Dijkstra's algorithm?Step size choice with l-bfgsIs there a fundamental concept underlying trade-offs in CS and are they unavoidable?Solving a Rod Cutting ProblemFeedback Vertex Set with vertex partitions?Does big-Oh notation in optimization follow the same convention as in CS?Big-O / $tildeO$ -notation with multiple variables when function is decreasing in one of its arguments
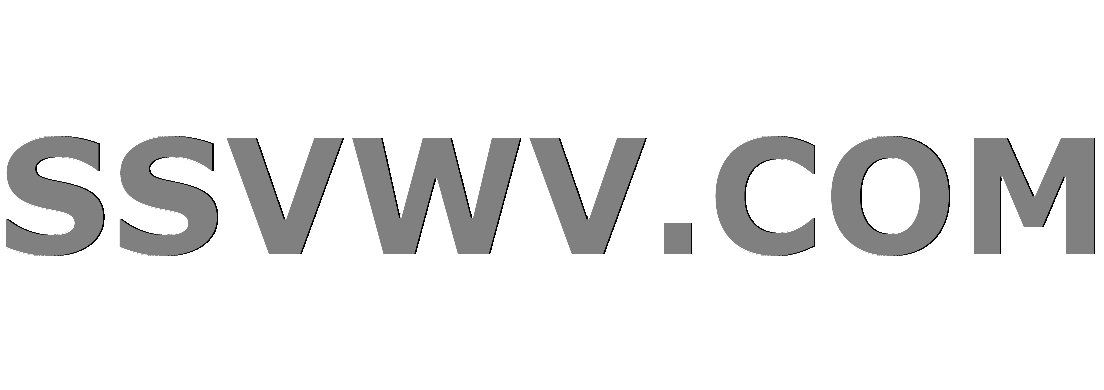
Multi tool use
Unary Enumeration
Local variables in DynamicModule affected by outside evaluation
What could be my risk mitigation strategies if my client wants to contract UAT?
Ribbon Cable Cross Talk - Is there a fix after the fact?
How to teach an undergraduate course without having taken that course formally before?
Why is 'additive' EQ more difficult to use than 'subtractive'?
Team has team lunch everyday, am I forced to go?
Alexandrov's generalization of Cauchy's rigidity theorem
How do you earn the reader's trust?
Why does Bran want to find Drogon?
To exponential digit growth and beyond!
Is this homebrew "Cactus Grenade" cantrip balanced?
Why isn't Tyrion mentioned in 'A song of Ice and Fire'?
Are cells guaranteed to get at least one mitochondrion when they divide?
"Official wife" or "Formal wife"?
Why did Drogon spare this character?
Gravitational Force Between Numbers
Why did it take so long for Germany to allow electric scooters / e-rollers on the roads?
Are PMR446 walkie-talkies legal in Switzerland?
What is Orcus doing with Mind Flayers in the art on the last page of Volo's Guide to Monsters?
Did Game of Thrones end the way that George RR Martin intended?
What is the purpose of the yellow wired panels on the IBM 360 Model 20?
Why did other houses not demand this?
Is it normal to "extract a paper" from a master thesis?
Are there variations of the regular runtimes of the Big-O-Notation?
For every computable function $f$ does there exist a problem that can be solved at best in $Theta(f(n))$ time?Progressive discrete multifunction optimizationInteger Programming - packing wolves and sheepHow to compute amortized complexity of n runs of Dijkstra's algorithm?Step size choice with l-bfgsIs there a fundamental concept underlying trade-offs in CS and are they unavoidable?Solving a Rod Cutting ProblemFeedback Vertex Set with vertex partitions?Does big-Oh notation in optimization follow the same convention as in CS?Big-O / $tildeO$ -notation with multiple variables when function is decreasing in one of its arguments
$begingroup$
There are multiple $O$-Notations, like $O(n)$ or $O(n^2)$ and so on. I was wondering, if there are variations of those in reality such as $O(2n^2)$ or $O(log n^2)$, or if those are mathematically incorrect.
Or would it be a right thing to say that it is possible to improve a $O(5n^2)$ to a $O(3n^2)$? I can't and don't need to figure out runtimes yet and I do not need to improve anything, but I'd need to know if this how you describe your functions in reality.
complexity-theory time-complexity optimization big-o-notation
$endgroup$
add a comment |
$begingroup$
There are multiple $O$-Notations, like $O(n)$ or $O(n^2)$ and so on. I was wondering, if there are variations of those in reality such as $O(2n^2)$ or $O(log n^2)$, or if those are mathematically incorrect.
Or would it be a right thing to say that it is possible to improve a $O(5n^2)$ to a $O(3n^2)$? I can't and don't need to figure out runtimes yet and I do not need to improve anything, but I'd need to know if this how you describe your functions in reality.
complexity-theory time-complexity optimization big-o-notation
$endgroup$
$begingroup$
There is no material difference between O(5n^2) to a O(3n^2) during an asymptotic analysis. They are both O(n^2), and only differ by a constant. In fact, in a proof, you might even reduce O(5n^2) to O(3n^2) or O(n^2) to make the math cleaner since they are equivalent. When writing your proof, you make a note in a sidebar that they are equivalent. In fact, you might even swap a O(log n) with O(n) and note that O(log n) <= O(n) in the sidebar. The note in the sidebar tells the reader it is intentional, and not a typo. (At least that's how I did it when I took Algorithm Analysis in college).
$endgroup$
– jww
May 9 at 20:54
2
$begingroup$
If you're using the $O(cdot)$ notation to get rid of small factors, you can always write something like "... improves the running time from $5n^2 + o(n^2)$ down to $3n^2 + o(n^2)$", etc. Or, equivalently, $(5 + o(1))n^2$ and $(3 + o(1))n^2$. Some authors prefer to just write $sim 5n^2$ as shorthand for the former. See, for example, the textbook by Trefethen and Bau.
$endgroup$
– Yonatan N
May 10 at 0:14
add a comment |
$begingroup$
There are multiple $O$-Notations, like $O(n)$ or $O(n^2)$ and so on. I was wondering, if there are variations of those in reality such as $O(2n^2)$ or $O(log n^2)$, or if those are mathematically incorrect.
Or would it be a right thing to say that it is possible to improve a $O(5n^2)$ to a $O(3n^2)$? I can't and don't need to figure out runtimes yet and I do not need to improve anything, but I'd need to know if this how you describe your functions in reality.
complexity-theory time-complexity optimization big-o-notation
$endgroup$
There are multiple $O$-Notations, like $O(n)$ or $O(n^2)$ and so on. I was wondering, if there are variations of those in reality such as $O(2n^2)$ or $O(log n^2)$, or if those are mathematically incorrect.
Or would it be a right thing to say that it is possible to improve a $O(5n^2)$ to a $O(3n^2)$? I can't and don't need to figure out runtimes yet and I do not need to improve anything, but I'd need to know if this how you describe your functions in reality.
complexity-theory time-complexity optimization big-o-notation
complexity-theory time-complexity optimization big-o-notation
edited May 9 at 7:54


Apass.Jack
16k11245
16k11245
asked May 9 at 7:49
bv_Martnbv_Martn
4614
4614
$begingroup$
There is no material difference between O(5n^2) to a O(3n^2) during an asymptotic analysis. They are both O(n^2), and only differ by a constant. In fact, in a proof, you might even reduce O(5n^2) to O(3n^2) or O(n^2) to make the math cleaner since they are equivalent. When writing your proof, you make a note in a sidebar that they are equivalent. In fact, you might even swap a O(log n) with O(n) and note that O(log n) <= O(n) in the sidebar. The note in the sidebar tells the reader it is intentional, and not a typo. (At least that's how I did it when I took Algorithm Analysis in college).
$endgroup$
– jww
May 9 at 20:54
2
$begingroup$
If you're using the $O(cdot)$ notation to get rid of small factors, you can always write something like "... improves the running time from $5n^2 + o(n^2)$ down to $3n^2 + o(n^2)$", etc. Or, equivalently, $(5 + o(1))n^2$ and $(3 + o(1))n^2$. Some authors prefer to just write $sim 5n^2$ as shorthand for the former. See, for example, the textbook by Trefethen and Bau.
$endgroup$
– Yonatan N
May 10 at 0:14
add a comment |
$begingroup$
There is no material difference between O(5n^2) to a O(3n^2) during an asymptotic analysis. They are both O(n^2), and only differ by a constant. In fact, in a proof, you might even reduce O(5n^2) to O(3n^2) or O(n^2) to make the math cleaner since they are equivalent. When writing your proof, you make a note in a sidebar that they are equivalent. In fact, you might even swap a O(log n) with O(n) and note that O(log n) <= O(n) in the sidebar. The note in the sidebar tells the reader it is intentional, and not a typo. (At least that's how I did it when I took Algorithm Analysis in college).
$endgroup$
– jww
May 9 at 20:54
2
$begingroup$
If you're using the $O(cdot)$ notation to get rid of small factors, you can always write something like "... improves the running time from $5n^2 + o(n^2)$ down to $3n^2 + o(n^2)$", etc. Or, equivalently, $(5 + o(1))n^2$ and $(3 + o(1))n^2$. Some authors prefer to just write $sim 5n^2$ as shorthand for the former. See, for example, the textbook by Trefethen and Bau.
$endgroup$
– Yonatan N
May 10 at 0:14
$begingroup$
There is no material difference between O(5n^2) to a O(3n^2) during an asymptotic analysis. They are both O(n^2), and only differ by a constant. In fact, in a proof, you might even reduce O(5n^2) to O(3n^2) or O(n^2) to make the math cleaner since they are equivalent. When writing your proof, you make a note in a sidebar that they are equivalent. In fact, you might even swap a O(log n) with O(n) and note that O(log n) <= O(n) in the sidebar. The note in the sidebar tells the reader it is intentional, and not a typo. (At least that's how I did it when I took Algorithm Analysis in college).
$endgroup$
– jww
May 9 at 20:54
$begingroup$
There is no material difference between O(5n^2) to a O(3n^2) during an asymptotic analysis. They are both O(n^2), and only differ by a constant. In fact, in a proof, you might even reduce O(5n^2) to O(3n^2) or O(n^2) to make the math cleaner since they are equivalent. When writing your proof, you make a note in a sidebar that they are equivalent. In fact, you might even swap a O(log n) with O(n) and note that O(log n) <= O(n) in the sidebar. The note in the sidebar tells the reader it is intentional, and not a typo. (At least that's how I did it when I took Algorithm Analysis in college).
$endgroup$
– jww
May 9 at 20:54
2
2
$begingroup$
If you're using the $O(cdot)$ notation to get rid of small factors, you can always write something like "... improves the running time from $5n^2 + o(n^2)$ down to $3n^2 + o(n^2)$", etc. Or, equivalently, $(5 + o(1))n^2$ and $(3 + o(1))n^2$. Some authors prefer to just write $sim 5n^2$ as shorthand for the former. See, for example, the textbook by Trefethen and Bau.
$endgroup$
– Yonatan N
May 10 at 0:14
$begingroup$
If you're using the $O(cdot)$ notation to get rid of small factors, you can always write something like "... improves the running time from $5n^2 + o(n^2)$ down to $3n^2 + o(n^2)$", etc. Or, equivalently, $(5 + o(1))n^2$ and $(3 + o(1))n^2$. Some authors prefer to just write $sim 5n^2$ as shorthand for the former. See, for example, the textbook by Trefethen and Bau.
$endgroup$
– Yonatan N
May 10 at 0:14
add a comment |
6 Answers
6
active
oldest
votes
$begingroup$
I was wondering, if there are variations of those in reality such as $O(2n^2)$ or $O(log (n^2))$, or if those are mathematically incorrect.
Yes, $O(2n^2)$ or $O(log (n^2))$ are valid variations.
However, you will see them rarely if you would see them at all, especially in the end results. The reason is that $O(2n^2)$ is $O(n^2)$. Similarly, $O(log (n^2))$ is $O(log n)$. That might be surprising to beginners. However, those equalities are more or less the very reason why big $O$-notations were introduced, to hide a multiplicative constant factor that is often hard to pin down and relatively insignificant.
Would it be a right thing to say that it is possible to improve a $O(5n^2)$ to a $O(3n^2)$?
It is not an improvement at all if the time-complexity of an algorithm is changed from $O(5n^2)$ to a $O(3n^2)$ or from $Omega(5n^2)$ to $Omega(3n^2)$, because $O(5n^2)$ is $O(3n^2)$ while $Omega(5n^2)$ is $Omega(3n^2)$. So it is incorrect to say the time-complexity is improved from $O(5n^2)$ to $O(3n^2)$. It is correct to say the time-complexity of an algorithm is improved from $5n^2$ to $3n^2$, of course.
Exercise 1. Show that $O(5n^2)=O(3n^2)=O(n^2)$.
Exercise 2. Show that $O(log n)=O(log (n^2))$.
Exercise 3. Show that $Omega(n^2+n)=Omega(n^2)$.
$endgroup$
1
$begingroup$
@bv_Martn Here's a good link to understand what the notation $O(n)$ is defined as (just simple limit calculus!): math.stackexchange.com/questions/925053/…
$endgroup$
– Akshat Mahajan
May 9 at 20:32
2
$begingroup$
The only time I've seen constant factors in big-O notation is when someone wants to make the point that, although two algorithms are of the same complexity class, one of them is strictly faster than the other.
$endgroup$
– Mark
May 9 at 20:42
7
$begingroup$
@AkshatMahajan The only answer to that question https://math.stackexchange.com/questions/925053 is plainly wrong. There are plenty of reliable sources on big $O$-notations.
$endgroup$
– Apass.Jack
May 9 at 20:56
1
$begingroup$
"It is correct to say the time-complexity of an algorithm is improved from 5n^2 to 3n^2" - although the exact running time often varies for different input sizes and values. Also, this involves weighting all operations / focusing on one operation, which may not say much about the constant factors you'll get in the real-world or be comparable to other algorithms using different weights. So, while it may have a few valid use cases, saying something like the above is of limited usefulness (which is probably why it's rarely seen).
$endgroup$
– Dukeling
May 10 at 8:14
1
$begingroup$
@Mark: That's simply wrong.
$endgroup$
– user21820
May 11 at 14:49
|
show 4 more comments
$begingroup$
You are always free to not use this notation at all. That is, you can determine a function $f(n)$ as precisely as possible, and then try to improve on that. For example, you might have a sorting algorithm that makes $f(n)$ comparisons, so you could try to come up with another sorting algorithm that only does $g(n)$ comparisons. Of course, all kinds of functions $f(n)$ exist (in theory) and can also come up (in practice).
Instead of treating the Big Oh notation as mysterious magic where you have to consult wizards to ask whether you can do something, you should look at its definition. Respect the definition, and then do whatever you need to get your job done.
$endgroup$
$begingroup$
Well I don't need it yet in practice. Or in theory actually, I just need to know if the wikipedia-given definitions O(1)-O(n!) are the only ones that exist, or if in reality you could describe them differently if they are different, such as O(7N). My fear is that if I use that a math professor will loose his wings
$endgroup$
– bv_Martn
May 9 at 8:00
1
$begingroup$
Any definition that anyone makes exists. You should read very carefully what the notation $O(1)$ or $O(n!)$ means because your question does not make sense. There are no shortcuts. If you want to understand what a piece of mathematical content means, you must be willing to invest some time.
$endgroup$
– Juho
May 9 at 8:14
6
$begingroup$
@bv_Martn The math professor is much more likely to flip out because you're viewing a list of examples as a list of definitions. So much of the point of mathematics is to define things in a way that makes them work generally, not just in specific cases. Your question is basically a more advanced version of "Wikipedia says that I can add one and add two and add seventeen. But can I add other numbers, too?"
$endgroup$
– David Richerby
May 9 at 9:22
add a comment |
$begingroup$
While the accepted answer is quite good, it still doesn't touch at the real reason why $O(n) = O(2n)$.
Big-O Notation describes scalability
At its core, Big-O Notation is not a description of how long an algorithm takes to run. Nor is it a description of how many steps, lines of code, or comparisons an algorithm makes. It is most useful when used to describe how an algorithm scales with the number of inputs.
Take a binary search, for example. Given a sorted list, how do you find an arbitrary value inside it? Well, you could start at the middle. Since the list is sorted, the middle value will tell you which half of the list your target value is in. So the list you have to search is now split in half. This can be applied recursively, then going to the middle of the new list, and so on until the list size is 1 and you've found your value (or it doesn't exist in the list). Doubling the size of the list only adds one extra step to the algorithm, which is a logarithmic relationship. Thus this algorithm is $O(log n)$. The logarithm is base 2, but that doesn't matter - the core of the relationship is that multiplying the list by a constant value only adds a constant value to the time.
Contrast a standard search through an unsorted list - the only way to search for a value in this case is to check each one. Worst-case scenario (which is what Big-O specifically implies) is that your value is at the very end, which means for a list of size $n$, you have to check $n$ values. Doubling the size of the list doubles the number of times you must check, which is a linear relationship. $O(n)$. But even if you had to perform two operations on each value, some processing, for example, the linear relationship still holds. $O(2n)$ simply isn't useful as a descriptor, since it would describe the exact same scalability as $O(n)$.
I appreciate that a lot of these answers are basically telling you to come to this conclusion yourself by reading the definition of Big-O. But this intuitive understanding took me quite a while to wrap my head around and so I lay it out to you as plainly as I can.
$endgroup$
5
$begingroup$
The biggest problem with this type of answer is that it does not touch on the definition of Big Oh, but just uses it as some sort of intuitive magic as in "see when you do this and this, it's $O(n)$". Personally, I think it's much more instructive to tell someone that Big Oh has absolutely nothing to do with algorithms necessarily and start with that.
$endgroup$
– Juho
May 9 at 13:42
3
$begingroup$
@Juho Instructive, maybe, but ultimately useless for the vast majority of computer scientists.
$endgroup$
– Christian
May 9 at 13:47
4
$begingroup$
With this I must disagree. Labeling oneself as a computer scientist should be no excuse for not understanding what a piece of notation one uses means, i.e., skipping all the math.
$endgroup$
– Juho
May 9 at 14:00
3
$begingroup$
Yeah. I've no objection to programmers not understanding this stuff but if you want to call yourself a computer scientist, then this is core material.
$endgroup$
– David Richerby
May 9 at 14:09
2
$begingroup$
@dkaeae No, I'm referring to people who work other careers in the field, such as software developers.
$endgroup$
– Christian
May 9 at 14:28
|
show 4 more comments
$begingroup$
You can write $O(f)$ for any function $f$ and it makes perfect sense. As per the definition, $g(n)=O(f(n))$ if there is some constant $c$ such that $g(n)leq c,f(n)$ for all large enough $n$. Nothing in that definition says that $f$ must be some sort of "nice" function.
But, as other answers have pointed out, $g(n)=O(f(n))$ and $g(n)=O(2f(n))$ describe exactly the same situation: if $g(n)leq c,f(n)$ for all all large enough $n$, then we also have $g(n)leq tfracc22f(n)$, so $g(n)=O(2f(n))$, also (taking the constant to be $c/2$).
As a side issue, don't write "$log n^2$", because it's not 100% clear what it means. You could say that it obviously means $log(n^2)$ but almost everybody would write that as $2log n$, so it puts doubt in the reader's mind.
Also, note that big-$O$ notation has nothing to do with runtimes per se. It's just a notation for relationships between functions. Those functions are often used to measure the runtimes of algorithms but that's just one application, just like measuring people's heights is just one application of numbers.
$endgroup$
add a comment |
$begingroup$
Look at the definition of O(f(n)), and you see that for example O(2n^2) and O(n^2) are exactly the same. Changing an algorithm from 5n^2 to 3n^2 operations is a 40 percent improvement. Changing from O(5n^2) to O(3n^2) isn’t actually any change, they are the same.
Again, read the definition of O(f(n)).
$endgroup$
add a comment |
$begingroup$
It may be helpful to understand that Big-O describes a set of functions. That is $O(f(n)) = lbrace g(n) | exists n,c>0: forall m > n: ctimes g(m) le f(m)rbrace$
The usage of $=$ is kind of unfortunate and using $in$ would make that relationship a lot clearer. but the set notation symbols are a bit difficult to type so now we are stuck with the current convention.
This then shows that $O(n) = O(2n)$ Or that constant factors don't matter when defining the Big O.
$endgroup$
4
$begingroup$
The equality convention isn't really about typing. It's because the usefulness of expressions such as $log (n!) = nlog n - n + O(log n)$ encourages us to view $O(f)$ as both "the set of functions such that [...]" and "some function such that [...]"
$endgroup$
– David Richerby
May 9 at 9:26
add a comment |
Your Answer
StackExchange.ready(function()
var channelOptions =
tags: "".split(" "),
id: "419"
;
initTagRenderer("".split(" "), "".split(" "), channelOptions);
StackExchange.using("externalEditor", function()
// Have to fire editor after snippets, if snippets enabled
if (StackExchange.settings.snippets.snippetsEnabled)
StackExchange.using("snippets", function()
createEditor();
);
else
createEditor();
);
function createEditor()
StackExchange.prepareEditor(
heartbeatType: 'answer',
autoActivateHeartbeat: false,
convertImagesToLinks: false,
noModals: true,
showLowRepImageUploadWarning: true,
reputationToPostImages: null,
bindNavPrevention: true,
postfix: "",
imageUploader:
brandingHtml: "Powered by u003ca class="icon-imgur-white" href="https://imgur.com/"u003eu003c/au003e",
contentPolicyHtml: "User contributions licensed under u003ca href="https://creativecommons.org/licenses/by-sa/3.0/"u003ecc by-sa 3.0 with attribution requiredu003c/au003e u003ca href="https://stackoverflow.com/legal/content-policy"u003e(content policy)u003c/au003e",
allowUrls: true
,
onDemand: true,
discardSelector: ".discard-answer"
,immediatelyShowMarkdownHelp:true
);
);
Sign up or log in
StackExchange.ready(function ()
StackExchange.helpers.onClickDraftSave('#login-link');
);
Sign up using Google
Sign up using Facebook
Sign up using Email and Password
Post as a guest
Required, but never shown
StackExchange.ready(
function ()
StackExchange.openid.initPostLogin('.new-post-login', 'https%3a%2f%2fcs.stackexchange.com%2fquestions%2f109127%2fare-there-variations-of-the-regular-runtimes-of-the-big-o-notation%23new-answer', 'question_page');
);
Post as a guest
Required, but never shown
6 Answers
6
active
oldest
votes
6 Answers
6
active
oldest
votes
active
oldest
votes
active
oldest
votes
$begingroup$
I was wondering, if there are variations of those in reality such as $O(2n^2)$ or $O(log (n^2))$, or if those are mathematically incorrect.
Yes, $O(2n^2)$ or $O(log (n^2))$ are valid variations.
However, you will see them rarely if you would see them at all, especially in the end results. The reason is that $O(2n^2)$ is $O(n^2)$. Similarly, $O(log (n^2))$ is $O(log n)$. That might be surprising to beginners. However, those equalities are more or less the very reason why big $O$-notations were introduced, to hide a multiplicative constant factor that is often hard to pin down and relatively insignificant.
Would it be a right thing to say that it is possible to improve a $O(5n^2)$ to a $O(3n^2)$?
It is not an improvement at all if the time-complexity of an algorithm is changed from $O(5n^2)$ to a $O(3n^2)$ or from $Omega(5n^2)$ to $Omega(3n^2)$, because $O(5n^2)$ is $O(3n^2)$ while $Omega(5n^2)$ is $Omega(3n^2)$. So it is incorrect to say the time-complexity is improved from $O(5n^2)$ to $O(3n^2)$. It is correct to say the time-complexity of an algorithm is improved from $5n^2$ to $3n^2$, of course.
Exercise 1. Show that $O(5n^2)=O(3n^2)=O(n^2)$.
Exercise 2. Show that $O(log n)=O(log (n^2))$.
Exercise 3. Show that $Omega(n^2+n)=Omega(n^2)$.
$endgroup$
1
$begingroup$
@bv_Martn Here's a good link to understand what the notation $O(n)$ is defined as (just simple limit calculus!): math.stackexchange.com/questions/925053/…
$endgroup$
– Akshat Mahajan
May 9 at 20:32
2
$begingroup$
The only time I've seen constant factors in big-O notation is when someone wants to make the point that, although two algorithms are of the same complexity class, one of them is strictly faster than the other.
$endgroup$
– Mark
May 9 at 20:42
7
$begingroup$
@AkshatMahajan The only answer to that question https://math.stackexchange.com/questions/925053 is plainly wrong. There are plenty of reliable sources on big $O$-notations.
$endgroup$
– Apass.Jack
May 9 at 20:56
1
$begingroup$
"It is correct to say the time-complexity of an algorithm is improved from 5n^2 to 3n^2" - although the exact running time often varies for different input sizes and values. Also, this involves weighting all operations / focusing on one operation, which may not say much about the constant factors you'll get in the real-world or be comparable to other algorithms using different weights. So, while it may have a few valid use cases, saying something like the above is of limited usefulness (which is probably why it's rarely seen).
$endgroup$
– Dukeling
May 10 at 8:14
1
$begingroup$
@Mark: That's simply wrong.
$endgroup$
– user21820
May 11 at 14:49
|
show 4 more comments
$begingroup$
I was wondering, if there are variations of those in reality such as $O(2n^2)$ or $O(log (n^2))$, or if those are mathematically incorrect.
Yes, $O(2n^2)$ or $O(log (n^2))$ are valid variations.
However, you will see them rarely if you would see them at all, especially in the end results. The reason is that $O(2n^2)$ is $O(n^2)$. Similarly, $O(log (n^2))$ is $O(log n)$. That might be surprising to beginners. However, those equalities are more or less the very reason why big $O$-notations were introduced, to hide a multiplicative constant factor that is often hard to pin down and relatively insignificant.
Would it be a right thing to say that it is possible to improve a $O(5n^2)$ to a $O(3n^2)$?
It is not an improvement at all if the time-complexity of an algorithm is changed from $O(5n^2)$ to a $O(3n^2)$ or from $Omega(5n^2)$ to $Omega(3n^2)$, because $O(5n^2)$ is $O(3n^2)$ while $Omega(5n^2)$ is $Omega(3n^2)$. So it is incorrect to say the time-complexity is improved from $O(5n^2)$ to $O(3n^2)$. It is correct to say the time-complexity of an algorithm is improved from $5n^2$ to $3n^2$, of course.
Exercise 1. Show that $O(5n^2)=O(3n^2)=O(n^2)$.
Exercise 2. Show that $O(log n)=O(log (n^2))$.
Exercise 3. Show that $Omega(n^2+n)=Omega(n^2)$.
$endgroup$
1
$begingroup$
@bv_Martn Here's a good link to understand what the notation $O(n)$ is defined as (just simple limit calculus!): math.stackexchange.com/questions/925053/…
$endgroup$
– Akshat Mahajan
May 9 at 20:32
2
$begingroup$
The only time I've seen constant factors in big-O notation is when someone wants to make the point that, although two algorithms are of the same complexity class, one of them is strictly faster than the other.
$endgroup$
– Mark
May 9 at 20:42
7
$begingroup$
@AkshatMahajan The only answer to that question https://math.stackexchange.com/questions/925053 is plainly wrong. There are plenty of reliable sources on big $O$-notations.
$endgroup$
– Apass.Jack
May 9 at 20:56
1
$begingroup$
"It is correct to say the time-complexity of an algorithm is improved from 5n^2 to 3n^2" - although the exact running time often varies for different input sizes and values. Also, this involves weighting all operations / focusing on one operation, which may not say much about the constant factors you'll get in the real-world or be comparable to other algorithms using different weights. So, while it may have a few valid use cases, saying something like the above is of limited usefulness (which is probably why it's rarely seen).
$endgroup$
– Dukeling
May 10 at 8:14
1
$begingroup$
@Mark: That's simply wrong.
$endgroup$
– user21820
May 11 at 14:49
|
show 4 more comments
$begingroup$
I was wondering, if there are variations of those in reality such as $O(2n^2)$ or $O(log (n^2))$, or if those are mathematically incorrect.
Yes, $O(2n^2)$ or $O(log (n^2))$ are valid variations.
However, you will see them rarely if you would see them at all, especially in the end results. The reason is that $O(2n^2)$ is $O(n^2)$. Similarly, $O(log (n^2))$ is $O(log n)$. That might be surprising to beginners. However, those equalities are more or less the very reason why big $O$-notations were introduced, to hide a multiplicative constant factor that is often hard to pin down and relatively insignificant.
Would it be a right thing to say that it is possible to improve a $O(5n^2)$ to a $O(3n^2)$?
It is not an improvement at all if the time-complexity of an algorithm is changed from $O(5n^2)$ to a $O(3n^2)$ or from $Omega(5n^2)$ to $Omega(3n^2)$, because $O(5n^2)$ is $O(3n^2)$ while $Omega(5n^2)$ is $Omega(3n^2)$. So it is incorrect to say the time-complexity is improved from $O(5n^2)$ to $O(3n^2)$. It is correct to say the time-complexity of an algorithm is improved from $5n^2$ to $3n^2$, of course.
Exercise 1. Show that $O(5n^2)=O(3n^2)=O(n^2)$.
Exercise 2. Show that $O(log n)=O(log (n^2))$.
Exercise 3. Show that $Omega(n^2+n)=Omega(n^2)$.
$endgroup$
I was wondering, if there are variations of those in reality such as $O(2n^2)$ or $O(log (n^2))$, or if those are mathematically incorrect.
Yes, $O(2n^2)$ or $O(log (n^2))$ are valid variations.
However, you will see them rarely if you would see them at all, especially in the end results. The reason is that $O(2n^2)$ is $O(n^2)$. Similarly, $O(log (n^2))$ is $O(log n)$. That might be surprising to beginners. However, those equalities are more or less the very reason why big $O$-notations were introduced, to hide a multiplicative constant factor that is often hard to pin down and relatively insignificant.
Would it be a right thing to say that it is possible to improve a $O(5n^2)$ to a $O(3n^2)$?
It is not an improvement at all if the time-complexity of an algorithm is changed from $O(5n^2)$ to a $O(3n^2)$ or from $Omega(5n^2)$ to $Omega(3n^2)$, because $O(5n^2)$ is $O(3n^2)$ while $Omega(5n^2)$ is $Omega(3n^2)$. So it is incorrect to say the time-complexity is improved from $O(5n^2)$ to $O(3n^2)$. It is correct to say the time-complexity of an algorithm is improved from $5n^2$ to $3n^2$, of course.
Exercise 1. Show that $O(5n^2)=O(3n^2)=O(n^2)$.
Exercise 2. Show that $O(log n)=O(log (n^2))$.
Exercise 3. Show that $Omega(n^2+n)=Omega(n^2)$.
edited May 9 at 22:53
eedrah
1033
1033
answered May 9 at 8:12


Apass.JackApass.Jack
16k11245
16k11245
1
$begingroup$
@bv_Martn Here's a good link to understand what the notation $O(n)$ is defined as (just simple limit calculus!): math.stackexchange.com/questions/925053/…
$endgroup$
– Akshat Mahajan
May 9 at 20:32
2
$begingroup$
The only time I've seen constant factors in big-O notation is when someone wants to make the point that, although two algorithms are of the same complexity class, one of them is strictly faster than the other.
$endgroup$
– Mark
May 9 at 20:42
7
$begingroup$
@AkshatMahajan The only answer to that question https://math.stackexchange.com/questions/925053 is plainly wrong. There are plenty of reliable sources on big $O$-notations.
$endgroup$
– Apass.Jack
May 9 at 20:56
1
$begingroup$
"It is correct to say the time-complexity of an algorithm is improved from 5n^2 to 3n^2" - although the exact running time often varies for different input sizes and values. Also, this involves weighting all operations / focusing on one operation, which may not say much about the constant factors you'll get in the real-world or be comparable to other algorithms using different weights. So, while it may have a few valid use cases, saying something like the above is of limited usefulness (which is probably why it's rarely seen).
$endgroup$
– Dukeling
May 10 at 8:14
1
$begingroup$
@Mark: That's simply wrong.
$endgroup$
– user21820
May 11 at 14:49
|
show 4 more comments
1
$begingroup$
@bv_Martn Here's a good link to understand what the notation $O(n)$ is defined as (just simple limit calculus!): math.stackexchange.com/questions/925053/…
$endgroup$
– Akshat Mahajan
May 9 at 20:32
2
$begingroup$
The only time I've seen constant factors in big-O notation is when someone wants to make the point that, although two algorithms are of the same complexity class, one of them is strictly faster than the other.
$endgroup$
– Mark
May 9 at 20:42
7
$begingroup$
@AkshatMahajan The only answer to that question https://math.stackexchange.com/questions/925053 is plainly wrong. There are plenty of reliable sources on big $O$-notations.
$endgroup$
– Apass.Jack
May 9 at 20:56
1
$begingroup$
"It is correct to say the time-complexity of an algorithm is improved from 5n^2 to 3n^2" - although the exact running time often varies for different input sizes and values. Also, this involves weighting all operations / focusing on one operation, which may not say much about the constant factors you'll get in the real-world or be comparable to other algorithms using different weights. So, while it may have a few valid use cases, saying something like the above is of limited usefulness (which is probably why it's rarely seen).
$endgroup$
– Dukeling
May 10 at 8:14
1
$begingroup$
@Mark: That's simply wrong.
$endgroup$
– user21820
May 11 at 14:49
1
1
$begingroup$
@bv_Martn Here's a good link to understand what the notation $O(n)$ is defined as (just simple limit calculus!): math.stackexchange.com/questions/925053/…
$endgroup$
– Akshat Mahajan
May 9 at 20:32
$begingroup$
@bv_Martn Here's a good link to understand what the notation $O(n)$ is defined as (just simple limit calculus!): math.stackexchange.com/questions/925053/…
$endgroup$
– Akshat Mahajan
May 9 at 20:32
2
2
$begingroup$
The only time I've seen constant factors in big-O notation is when someone wants to make the point that, although two algorithms are of the same complexity class, one of them is strictly faster than the other.
$endgroup$
– Mark
May 9 at 20:42
$begingroup$
The only time I've seen constant factors in big-O notation is when someone wants to make the point that, although two algorithms are of the same complexity class, one of them is strictly faster than the other.
$endgroup$
– Mark
May 9 at 20:42
7
7
$begingroup$
@AkshatMahajan The only answer to that question https://math.stackexchange.com/questions/925053 is plainly wrong. There are plenty of reliable sources on big $O$-notations.
$endgroup$
– Apass.Jack
May 9 at 20:56
$begingroup$
@AkshatMahajan The only answer to that question https://math.stackexchange.com/questions/925053 is plainly wrong. There are plenty of reliable sources on big $O$-notations.
$endgroup$
– Apass.Jack
May 9 at 20:56
1
1
$begingroup$
"It is correct to say the time-complexity of an algorithm is improved from 5n^2 to 3n^2" - although the exact running time often varies for different input sizes and values. Also, this involves weighting all operations / focusing on one operation, which may not say much about the constant factors you'll get in the real-world or be comparable to other algorithms using different weights. So, while it may have a few valid use cases, saying something like the above is of limited usefulness (which is probably why it's rarely seen).
$endgroup$
– Dukeling
May 10 at 8:14
$begingroup$
"It is correct to say the time-complexity of an algorithm is improved from 5n^2 to 3n^2" - although the exact running time often varies for different input sizes and values. Also, this involves weighting all operations / focusing on one operation, which may not say much about the constant factors you'll get in the real-world or be comparable to other algorithms using different weights. So, while it may have a few valid use cases, saying something like the above is of limited usefulness (which is probably why it's rarely seen).
$endgroup$
– Dukeling
May 10 at 8:14
1
1
$begingroup$
@Mark: That's simply wrong.
$endgroup$
– user21820
May 11 at 14:49
$begingroup$
@Mark: That's simply wrong.
$endgroup$
– user21820
May 11 at 14:49
|
show 4 more comments
$begingroup$
You are always free to not use this notation at all. That is, you can determine a function $f(n)$ as precisely as possible, and then try to improve on that. For example, you might have a sorting algorithm that makes $f(n)$ comparisons, so you could try to come up with another sorting algorithm that only does $g(n)$ comparisons. Of course, all kinds of functions $f(n)$ exist (in theory) and can also come up (in practice).
Instead of treating the Big Oh notation as mysterious magic where you have to consult wizards to ask whether you can do something, you should look at its definition. Respect the definition, and then do whatever you need to get your job done.
$endgroup$
$begingroup$
Well I don't need it yet in practice. Or in theory actually, I just need to know if the wikipedia-given definitions O(1)-O(n!) are the only ones that exist, or if in reality you could describe them differently if they are different, such as O(7N). My fear is that if I use that a math professor will loose his wings
$endgroup$
– bv_Martn
May 9 at 8:00
1
$begingroup$
Any definition that anyone makes exists. You should read very carefully what the notation $O(1)$ or $O(n!)$ means because your question does not make sense. There are no shortcuts. If you want to understand what a piece of mathematical content means, you must be willing to invest some time.
$endgroup$
– Juho
May 9 at 8:14
6
$begingroup$
@bv_Martn The math professor is much more likely to flip out because you're viewing a list of examples as a list of definitions. So much of the point of mathematics is to define things in a way that makes them work generally, not just in specific cases. Your question is basically a more advanced version of "Wikipedia says that I can add one and add two and add seventeen. But can I add other numbers, too?"
$endgroup$
– David Richerby
May 9 at 9:22
add a comment |
$begingroup$
You are always free to not use this notation at all. That is, you can determine a function $f(n)$ as precisely as possible, and then try to improve on that. For example, you might have a sorting algorithm that makes $f(n)$ comparisons, so you could try to come up with another sorting algorithm that only does $g(n)$ comparisons. Of course, all kinds of functions $f(n)$ exist (in theory) and can also come up (in practice).
Instead of treating the Big Oh notation as mysterious magic where you have to consult wizards to ask whether you can do something, you should look at its definition. Respect the definition, and then do whatever you need to get your job done.
$endgroup$
$begingroup$
Well I don't need it yet in practice. Or in theory actually, I just need to know if the wikipedia-given definitions O(1)-O(n!) are the only ones that exist, or if in reality you could describe them differently if they are different, such as O(7N). My fear is that if I use that a math professor will loose his wings
$endgroup$
– bv_Martn
May 9 at 8:00
1
$begingroup$
Any definition that anyone makes exists. You should read very carefully what the notation $O(1)$ or $O(n!)$ means because your question does not make sense. There are no shortcuts. If you want to understand what a piece of mathematical content means, you must be willing to invest some time.
$endgroup$
– Juho
May 9 at 8:14
6
$begingroup$
@bv_Martn The math professor is much more likely to flip out because you're viewing a list of examples as a list of definitions. So much of the point of mathematics is to define things in a way that makes them work generally, not just in specific cases. Your question is basically a more advanced version of "Wikipedia says that I can add one and add two and add seventeen. But can I add other numbers, too?"
$endgroup$
– David Richerby
May 9 at 9:22
add a comment |
$begingroup$
You are always free to not use this notation at all. That is, you can determine a function $f(n)$ as precisely as possible, and then try to improve on that. For example, you might have a sorting algorithm that makes $f(n)$ comparisons, so you could try to come up with another sorting algorithm that only does $g(n)$ comparisons. Of course, all kinds of functions $f(n)$ exist (in theory) and can also come up (in practice).
Instead of treating the Big Oh notation as mysterious magic where you have to consult wizards to ask whether you can do something, you should look at its definition. Respect the definition, and then do whatever you need to get your job done.
$endgroup$
You are always free to not use this notation at all. That is, you can determine a function $f(n)$ as precisely as possible, and then try to improve on that. For example, you might have a sorting algorithm that makes $f(n)$ comparisons, so you could try to come up with another sorting algorithm that only does $g(n)$ comparisons. Of course, all kinds of functions $f(n)$ exist (in theory) and can also come up (in practice).
Instead of treating the Big Oh notation as mysterious magic where you have to consult wizards to ask whether you can do something, you should look at its definition. Respect the definition, and then do whatever you need to get your job done.
answered May 9 at 7:56
JuhoJuho
16.2k54293
16.2k54293
$begingroup$
Well I don't need it yet in practice. Or in theory actually, I just need to know if the wikipedia-given definitions O(1)-O(n!) are the only ones that exist, or if in reality you could describe them differently if they are different, such as O(7N). My fear is that if I use that a math professor will loose his wings
$endgroup$
– bv_Martn
May 9 at 8:00
1
$begingroup$
Any definition that anyone makes exists. You should read very carefully what the notation $O(1)$ or $O(n!)$ means because your question does not make sense. There are no shortcuts. If you want to understand what a piece of mathematical content means, you must be willing to invest some time.
$endgroup$
– Juho
May 9 at 8:14
6
$begingroup$
@bv_Martn The math professor is much more likely to flip out because you're viewing a list of examples as a list of definitions. So much of the point of mathematics is to define things in a way that makes them work generally, not just in specific cases. Your question is basically a more advanced version of "Wikipedia says that I can add one and add two and add seventeen. But can I add other numbers, too?"
$endgroup$
– David Richerby
May 9 at 9:22
add a comment |
$begingroup$
Well I don't need it yet in practice. Or in theory actually, I just need to know if the wikipedia-given definitions O(1)-O(n!) are the only ones that exist, or if in reality you could describe them differently if they are different, such as O(7N). My fear is that if I use that a math professor will loose his wings
$endgroup$
– bv_Martn
May 9 at 8:00
1
$begingroup$
Any definition that anyone makes exists. You should read very carefully what the notation $O(1)$ or $O(n!)$ means because your question does not make sense. There are no shortcuts. If you want to understand what a piece of mathematical content means, you must be willing to invest some time.
$endgroup$
– Juho
May 9 at 8:14
6
$begingroup$
@bv_Martn The math professor is much more likely to flip out because you're viewing a list of examples as a list of definitions. So much of the point of mathematics is to define things in a way that makes them work generally, not just in specific cases. Your question is basically a more advanced version of "Wikipedia says that I can add one and add two and add seventeen. But can I add other numbers, too?"
$endgroup$
– David Richerby
May 9 at 9:22
$begingroup$
Well I don't need it yet in practice. Or in theory actually, I just need to know if the wikipedia-given definitions O(1)-O(n!) are the only ones that exist, or if in reality you could describe them differently if they are different, such as O(7N). My fear is that if I use that a math professor will loose his wings
$endgroup$
– bv_Martn
May 9 at 8:00
$begingroup$
Well I don't need it yet in practice. Or in theory actually, I just need to know if the wikipedia-given definitions O(1)-O(n!) are the only ones that exist, or if in reality you could describe them differently if they are different, such as O(7N). My fear is that if I use that a math professor will loose his wings
$endgroup$
– bv_Martn
May 9 at 8:00
1
1
$begingroup$
Any definition that anyone makes exists. You should read very carefully what the notation $O(1)$ or $O(n!)$ means because your question does not make sense. There are no shortcuts. If you want to understand what a piece of mathematical content means, you must be willing to invest some time.
$endgroup$
– Juho
May 9 at 8:14
$begingroup$
Any definition that anyone makes exists. You should read very carefully what the notation $O(1)$ or $O(n!)$ means because your question does not make sense. There are no shortcuts. If you want to understand what a piece of mathematical content means, you must be willing to invest some time.
$endgroup$
– Juho
May 9 at 8:14
6
6
$begingroup$
@bv_Martn The math professor is much more likely to flip out because you're viewing a list of examples as a list of definitions. So much of the point of mathematics is to define things in a way that makes them work generally, not just in specific cases. Your question is basically a more advanced version of "Wikipedia says that I can add one and add two and add seventeen. But can I add other numbers, too?"
$endgroup$
– David Richerby
May 9 at 9:22
$begingroup$
@bv_Martn The math professor is much more likely to flip out because you're viewing a list of examples as a list of definitions. So much of the point of mathematics is to define things in a way that makes them work generally, not just in specific cases. Your question is basically a more advanced version of "Wikipedia says that I can add one and add two and add seventeen. But can I add other numbers, too?"
$endgroup$
– David Richerby
May 9 at 9:22
add a comment |
$begingroup$
While the accepted answer is quite good, it still doesn't touch at the real reason why $O(n) = O(2n)$.
Big-O Notation describes scalability
At its core, Big-O Notation is not a description of how long an algorithm takes to run. Nor is it a description of how many steps, lines of code, or comparisons an algorithm makes. It is most useful when used to describe how an algorithm scales with the number of inputs.
Take a binary search, for example. Given a sorted list, how do you find an arbitrary value inside it? Well, you could start at the middle. Since the list is sorted, the middle value will tell you which half of the list your target value is in. So the list you have to search is now split in half. This can be applied recursively, then going to the middle of the new list, and so on until the list size is 1 and you've found your value (or it doesn't exist in the list). Doubling the size of the list only adds one extra step to the algorithm, which is a logarithmic relationship. Thus this algorithm is $O(log n)$. The logarithm is base 2, but that doesn't matter - the core of the relationship is that multiplying the list by a constant value only adds a constant value to the time.
Contrast a standard search through an unsorted list - the only way to search for a value in this case is to check each one. Worst-case scenario (which is what Big-O specifically implies) is that your value is at the very end, which means for a list of size $n$, you have to check $n$ values. Doubling the size of the list doubles the number of times you must check, which is a linear relationship. $O(n)$. But even if you had to perform two operations on each value, some processing, for example, the linear relationship still holds. $O(2n)$ simply isn't useful as a descriptor, since it would describe the exact same scalability as $O(n)$.
I appreciate that a lot of these answers are basically telling you to come to this conclusion yourself by reading the definition of Big-O. But this intuitive understanding took me quite a while to wrap my head around and so I lay it out to you as plainly as I can.
$endgroup$
5
$begingroup$
The biggest problem with this type of answer is that it does not touch on the definition of Big Oh, but just uses it as some sort of intuitive magic as in "see when you do this and this, it's $O(n)$". Personally, I think it's much more instructive to tell someone that Big Oh has absolutely nothing to do with algorithms necessarily and start with that.
$endgroup$
– Juho
May 9 at 13:42
3
$begingroup$
@Juho Instructive, maybe, but ultimately useless for the vast majority of computer scientists.
$endgroup$
– Christian
May 9 at 13:47
4
$begingroup$
With this I must disagree. Labeling oneself as a computer scientist should be no excuse for not understanding what a piece of notation one uses means, i.e., skipping all the math.
$endgroup$
– Juho
May 9 at 14:00
3
$begingroup$
Yeah. I've no objection to programmers not understanding this stuff but if you want to call yourself a computer scientist, then this is core material.
$endgroup$
– David Richerby
May 9 at 14:09
2
$begingroup$
@dkaeae No, I'm referring to people who work other careers in the field, such as software developers.
$endgroup$
– Christian
May 9 at 14:28
|
show 4 more comments
$begingroup$
While the accepted answer is quite good, it still doesn't touch at the real reason why $O(n) = O(2n)$.
Big-O Notation describes scalability
At its core, Big-O Notation is not a description of how long an algorithm takes to run. Nor is it a description of how many steps, lines of code, or comparisons an algorithm makes. It is most useful when used to describe how an algorithm scales with the number of inputs.
Take a binary search, for example. Given a sorted list, how do you find an arbitrary value inside it? Well, you could start at the middle. Since the list is sorted, the middle value will tell you which half of the list your target value is in. So the list you have to search is now split in half. This can be applied recursively, then going to the middle of the new list, and so on until the list size is 1 and you've found your value (or it doesn't exist in the list). Doubling the size of the list only adds one extra step to the algorithm, which is a logarithmic relationship. Thus this algorithm is $O(log n)$. The logarithm is base 2, but that doesn't matter - the core of the relationship is that multiplying the list by a constant value only adds a constant value to the time.
Contrast a standard search through an unsorted list - the only way to search for a value in this case is to check each one. Worst-case scenario (which is what Big-O specifically implies) is that your value is at the very end, which means for a list of size $n$, you have to check $n$ values. Doubling the size of the list doubles the number of times you must check, which is a linear relationship. $O(n)$. But even if you had to perform two operations on each value, some processing, for example, the linear relationship still holds. $O(2n)$ simply isn't useful as a descriptor, since it would describe the exact same scalability as $O(n)$.
I appreciate that a lot of these answers are basically telling you to come to this conclusion yourself by reading the definition of Big-O. But this intuitive understanding took me quite a while to wrap my head around and so I lay it out to you as plainly as I can.
$endgroup$
5
$begingroup$
The biggest problem with this type of answer is that it does not touch on the definition of Big Oh, but just uses it as some sort of intuitive magic as in "see when you do this and this, it's $O(n)$". Personally, I think it's much more instructive to tell someone that Big Oh has absolutely nothing to do with algorithms necessarily and start with that.
$endgroup$
– Juho
May 9 at 13:42
3
$begingroup$
@Juho Instructive, maybe, but ultimately useless for the vast majority of computer scientists.
$endgroup$
– Christian
May 9 at 13:47
4
$begingroup$
With this I must disagree. Labeling oneself as a computer scientist should be no excuse for not understanding what a piece of notation one uses means, i.e., skipping all the math.
$endgroup$
– Juho
May 9 at 14:00
3
$begingroup$
Yeah. I've no objection to programmers not understanding this stuff but if you want to call yourself a computer scientist, then this is core material.
$endgroup$
– David Richerby
May 9 at 14:09
2
$begingroup$
@dkaeae No, I'm referring to people who work other careers in the field, such as software developers.
$endgroup$
– Christian
May 9 at 14:28
|
show 4 more comments
$begingroup$
While the accepted answer is quite good, it still doesn't touch at the real reason why $O(n) = O(2n)$.
Big-O Notation describes scalability
At its core, Big-O Notation is not a description of how long an algorithm takes to run. Nor is it a description of how many steps, lines of code, or comparisons an algorithm makes. It is most useful when used to describe how an algorithm scales with the number of inputs.
Take a binary search, for example. Given a sorted list, how do you find an arbitrary value inside it? Well, you could start at the middle. Since the list is sorted, the middle value will tell you which half of the list your target value is in. So the list you have to search is now split in half. This can be applied recursively, then going to the middle of the new list, and so on until the list size is 1 and you've found your value (or it doesn't exist in the list). Doubling the size of the list only adds one extra step to the algorithm, which is a logarithmic relationship. Thus this algorithm is $O(log n)$. The logarithm is base 2, but that doesn't matter - the core of the relationship is that multiplying the list by a constant value only adds a constant value to the time.
Contrast a standard search through an unsorted list - the only way to search for a value in this case is to check each one. Worst-case scenario (which is what Big-O specifically implies) is that your value is at the very end, which means for a list of size $n$, you have to check $n$ values. Doubling the size of the list doubles the number of times you must check, which is a linear relationship. $O(n)$. But even if you had to perform two operations on each value, some processing, for example, the linear relationship still holds. $O(2n)$ simply isn't useful as a descriptor, since it would describe the exact same scalability as $O(n)$.
I appreciate that a lot of these answers are basically telling you to come to this conclusion yourself by reading the definition of Big-O. But this intuitive understanding took me quite a while to wrap my head around and so I lay it out to you as plainly as I can.
$endgroup$
While the accepted answer is quite good, it still doesn't touch at the real reason why $O(n) = O(2n)$.
Big-O Notation describes scalability
At its core, Big-O Notation is not a description of how long an algorithm takes to run. Nor is it a description of how many steps, lines of code, or comparisons an algorithm makes. It is most useful when used to describe how an algorithm scales with the number of inputs.
Take a binary search, for example. Given a sorted list, how do you find an arbitrary value inside it? Well, you could start at the middle. Since the list is sorted, the middle value will tell you which half of the list your target value is in. So the list you have to search is now split in half. This can be applied recursively, then going to the middle of the new list, and so on until the list size is 1 and you've found your value (or it doesn't exist in the list). Doubling the size of the list only adds one extra step to the algorithm, which is a logarithmic relationship. Thus this algorithm is $O(log n)$. The logarithm is base 2, but that doesn't matter - the core of the relationship is that multiplying the list by a constant value only adds a constant value to the time.
Contrast a standard search through an unsorted list - the only way to search for a value in this case is to check each one. Worst-case scenario (which is what Big-O specifically implies) is that your value is at the very end, which means for a list of size $n$, you have to check $n$ values. Doubling the size of the list doubles the number of times you must check, which is a linear relationship. $O(n)$. But even if you had to perform two operations on each value, some processing, for example, the linear relationship still holds. $O(2n)$ simply isn't useful as a descriptor, since it would describe the exact same scalability as $O(n)$.
I appreciate that a lot of these answers are basically telling you to come to this conclusion yourself by reading the definition of Big-O. But this intuitive understanding took me quite a while to wrap my head around and so I lay it out to you as plainly as I can.
answered May 9 at 13:35
ChristianChristian
1711
1711
5
$begingroup$
The biggest problem with this type of answer is that it does not touch on the definition of Big Oh, but just uses it as some sort of intuitive magic as in "see when you do this and this, it's $O(n)$". Personally, I think it's much more instructive to tell someone that Big Oh has absolutely nothing to do with algorithms necessarily and start with that.
$endgroup$
– Juho
May 9 at 13:42
3
$begingroup$
@Juho Instructive, maybe, but ultimately useless for the vast majority of computer scientists.
$endgroup$
– Christian
May 9 at 13:47
4
$begingroup$
With this I must disagree. Labeling oneself as a computer scientist should be no excuse for not understanding what a piece of notation one uses means, i.e., skipping all the math.
$endgroup$
– Juho
May 9 at 14:00
3
$begingroup$
Yeah. I've no objection to programmers not understanding this stuff but if you want to call yourself a computer scientist, then this is core material.
$endgroup$
– David Richerby
May 9 at 14:09
2
$begingroup$
@dkaeae No, I'm referring to people who work other careers in the field, such as software developers.
$endgroup$
– Christian
May 9 at 14:28
|
show 4 more comments
5
$begingroup$
The biggest problem with this type of answer is that it does not touch on the definition of Big Oh, but just uses it as some sort of intuitive magic as in "see when you do this and this, it's $O(n)$". Personally, I think it's much more instructive to tell someone that Big Oh has absolutely nothing to do with algorithms necessarily and start with that.
$endgroup$
– Juho
May 9 at 13:42
3
$begingroup$
@Juho Instructive, maybe, but ultimately useless for the vast majority of computer scientists.
$endgroup$
– Christian
May 9 at 13:47
4
$begingroup$
With this I must disagree. Labeling oneself as a computer scientist should be no excuse for not understanding what a piece of notation one uses means, i.e., skipping all the math.
$endgroup$
– Juho
May 9 at 14:00
3
$begingroup$
Yeah. I've no objection to programmers not understanding this stuff but if you want to call yourself a computer scientist, then this is core material.
$endgroup$
– David Richerby
May 9 at 14:09
2
$begingroup$
@dkaeae No, I'm referring to people who work other careers in the field, such as software developers.
$endgroup$
– Christian
May 9 at 14:28
5
5
$begingroup$
The biggest problem with this type of answer is that it does not touch on the definition of Big Oh, but just uses it as some sort of intuitive magic as in "see when you do this and this, it's $O(n)$". Personally, I think it's much more instructive to tell someone that Big Oh has absolutely nothing to do with algorithms necessarily and start with that.
$endgroup$
– Juho
May 9 at 13:42
$begingroup$
The biggest problem with this type of answer is that it does not touch on the definition of Big Oh, but just uses it as some sort of intuitive magic as in "see when you do this and this, it's $O(n)$". Personally, I think it's much more instructive to tell someone that Big Oh has absolutely nothing to do with algorithms necessarily and start with that.
$endgroup$
– Juho
May 9 at 13:42
3
3
$begingroup$
@Juho Instructive, maybe, but ultimately useless for the vast majority of computer scientists.
$endgroup$
– Christian
May 9 at 13:47
$begingroup$
@Juho Instructive, maybe, but ultimately useless for the vast majority of computer scientists.
$endgroup$
– Christian
May 9 at 13:47
4
4
$begingroup$
With this I must disagree. Labeling oneself as a computer scientist should be no excuse for not understanding what a piece of notation one uses means, i.e., skipping all the math.
$endgroup$
– Juho
May 9 at 14:00
$begingroup$
With this I must disagree. Labeling oneself as a computer scientist should be no excuse for not understanding what a piece of notation one uses means, i.e., skipping all the math.
$endgroup$
– Juho
May 9 at 14:00
3
3
$begingroup$
Yeah. I've no objection to programmers not understanding this stuff but if you want to call yourself a computer scientist, then this is core material.
$endgroup$
– David Richerby
May 9 at 14:09
$begingroup$
Yeah. I've no objection to programmers not understanding this stuff but if you want to call yourself a computer scientist, then this is core material.
$endgroup$
– David Richerby
May 9 at 14:09
2
2
$begingroup$
@dkaeae No, I'm referring to people who work other careers in the field, such as software developers.
$endgroup$
– Christian
May 9 at 14:28
$begingroup$
@dkaeae No, I'm referring to people who work other careers in the field, such as software developers.
$endgroup$
– Christian
May 9 at 14:28
|
show 4 more comments
$begingroup$
You can write $O(f)$ for any function $f$ and it makes perfect sense. As per the definition, $g(n)=O(f(n))$ if there is some constant $c$ such that $g(n)leq c,f(n)$ for all large enough $n$. Nothing in that definition says that $f$ must be some sort of "nice" function.
But, as other answers have pointed out, $g(n)=O(f(n))$ and $g(n)=O(2f(n))$ describe exactly the same situation: if $g(n)leq c,f(n)$ for all all large enough $n$, then we also have $g(n)leq tfracc22f(n)$, so $g(n)=O(2f(n))$, also (taking the constant to be $c/2$).
As a side issue, don't write "$log n^2$", because it's not 100% clear what it means. You could say that it obviously means $log(n^2)$ but almost everybody would write that as $2log n$, so it puts doubt in the reader's mind.
Also, note that big-$O$ notation has nothing to do with runtimes per se. It's just a notation for relationships between functions. Those functions are often used to measure the runtimes of algorithms but that's just one application, just like measuring people's heights is just one application of numbers.
$endgroup$
add a comment |
$begingroup$
You can write $O(f)$ for any function $f$ and it makes perfect sense. As per the definition, $g(n)=O(f(n))$ if there is some constant $c$ such that $g(n)leq c,f(n)$ for all large enough $n$. Nothing in that definition says that $f$ must be some sort of "nice" function.
But, as other answers have pointed out, $g(n)=O(f(n))$ and $g(n)=O(2f(n))$ describe exactly the same situation: if $g(n)leq c,f(n)$ for all all large enough $n$, then we also have $g(n)leq tfracc22f(n)$, so $g(n)=O(2f(n))$, also (taking the constant to be $c/2$).
As a side issue, don't write "$log n^2$", because it's not 100% clear what it means. You could say that it obviously means $log(n^2)$ but almost everybody would write that as $2log n$, so it puts doubt in the reader's mind.
Also, note that big-$O$ notation has nothing to do with runtimes per se. It's just a notation for relationships between functions. Those functions are often used to measure the runtimes of algorithms but that's just one application, just like measuring people's heights is just one application of numbers.
$endgroup$
add a comment |
$begingroup$
You can write $O(f)$ for any function $f$ and it makes perfect sense. As per the definition, $g(n)=O(f(n))$ if there is some constant $c$ such that $g(n)leq c,f(n)$ for all large enough $n$. Nothing in that definition says that $f$ must be some sort of "nice" function.
But, as other answers have pointed out, $g(n)=O(f(n))$ and $g(n)=O(2f(n))$ describe exactly the same situation: if $g(n)leq c,f(n)$ for all all large enough $n$, then we also have $g(n)leq tfracc22f(n)$, so $g(n)=O(2f(n))$, also (taking the constant to be $c/2$).
As a side issue, don't write "$log n^2$", because it's not 100% clear what it means. You could say that it obviously means $log(n^2)$ but almost everybody would write that as $2log n$, so it puts doubt in the reader's mind.
Also, note that big-$O$ notation has nothing to do with runtimes per se. It's just a notation for relationships between functions. Those functions are often used to measure the runtimes of algorithms but that's just one application, just like measuring people's heights is just one application of numbers.
$endgroup$
You can write $O(f)$ for any function $f$ and it makes perfect sense. As per the definition, $g(n)=O(f(n))$ if there is some constant $c$ such that $g(n)leq c,f(n)$ for all large enough $n$. Nothing in that definition says that $f$ must be some sort of "nice" function.
But, as other answers have pointed out, $g(n)=O(f(n))$ and $g(n)=O(2f(n))$ describe exactly the same situation: if $g(n)leq c,f(n)$ for all all large enough $n$, then we also have $g(n)leq tfracc22f(n)$, so $g(n)=O(2f(n))$, also (taking the constant to be $c/2$).
As a side issue, don't write "$log n^2$", because it's not 100% clear what it means. You could say that it obviously means $log(n^2)$ but almost everybody would write that as $2log n$, so it puts doubt in the reader's mind.
Also, note that big-$O$ notation has nothing to do with runtimes per se. It's just a notation for relationships between functions. Those functions are often used to measure the runtimes of algorithms but that's just one application, just like measuring people's heights is just one application of numbers.
edited May 9 at 9:28
answered May 9 at 9:20


David RicherbyDavid Richerby
72.2k16111201
72.2k16111201
add a comment |
add a comment |
$begingroup$
Look at the definition of O(f(n)), and you see that for example O(2n^2) and O(n^2) are exactly the same. Changing an algorithm from 5n^2 to 3n^2 operations is a 40 percent improvement. Changing from O(5n^2) to O(3n^2) isn’t actually any change, they are the same.
Again, read the definition of O(f(n)).
$endgroup$
add a comment |
$begingroup$
Look at the definition of O(f(n)), and you see that for example O(2n^2) and O(n^2) are exactly the same. Changing an algorithm from 5n^2 to 3n^2 operations is a 40 percent improvement. Changing from O(5n^2) to O(3n^2) isn’t actually any change, they are the same.
Again, read the definition of O(f(n)).
$endgroup$
add a comment |
$begingroup$
Look at the definition of O(f(n)), and you see that for example O(2n^2) and O(n^2) are exactly the same. Changing an algorithm from 5n^2 to 3n^2 operations is a 40 percent improvement. Changing from O(5n^2) to O(3n^2) isn’t actually any change, they are the same.
Again, read the definition of O(f(n)).
$endgroup$
Look at the definition of O(f(n)), and you see that for example O(2n^2) and O(n^2) are exactly the same. Changing an algorithm from 5n^2 to 3n^2 operations is a 40 percent improvement. Changing from O(5n^2) to O(3n^2) isn’t actually any change, they are the same.
Again, read the definition of O(f(n)).
answered May 9 at 8:10
gnasher729gnasher729
13k1623
13k1623
add a comment |
add a comment |
$begingroup$
It may be helpful to understand that Big-O describes a set of functions. That is $O(f(n)) = lbrace g(n) | exists n,c>0: forall m > n: ctimes g(m) le f(m)rbrace$
The usage of $=$ is kind of unfortunate and using $in$ would make that relationship a lot clearer. but the set notation symbols are a bit difficult to type so now we are stuck with the current convention.
This then shows that $O(n) = O(2n)$ Or that constant factors don't matter when defining the Big O.
$endgroup$
4
$begingroup$
The equality convention isn't really about typing. It's because the usefulness of expressions such as $log (n!) = nlog n - n + O(log n)$ encourages us to view $O(f)$ as both "the set of functions such that [...]" and "some function such that [...]"
$endgroup$
– David Richerby
May 9 at 9:26
add a comment |
$begingroup$
It may be helpful to understand that Big-O describes a set of functions. That is $O(f(n)) = lbrace g(n) | exists n,c>0: forall m > n: ctimes g(m) le f(m)rbrace$
The usage of $=$ is kind of unfortunate and using $in$ would make that relationship a lot clearer. but the set notation symbols are a bit difficult to type so now we are stuck with the current convention.
This then shows that $O(n) = O(2n)$ Or that constant factors don't matter when defining the Big O.
$endgroup$
4
$begingroup$
The equality convention isn't really about typing. It's because the usefulness of expressions such as $log (n!) = nlog n - n + O(log n)$ encourages us to view $O(f)$ as both "the set of functions such that [...]" and "some function such that [...]"
$endgroup$
– David Richerby
May 9 at 9:26
add a comment |
$begingroup$
It may be helpful to understand that Big-O describes a set of functions. That is $O(f(n)) = lbrace g(n) | exists n,c>0: forall m > n: ctimes g(m) le f(m)rbrace$
The usage of $=$ is kind of unfortunate and using $in$ would make that relationship a lot clearer. but the set notation symbols are a bit difficult to type so now we are stuck with the current convention.
This then shows that $O(n) = O(2n)$ Or that constant factors don't matter when defining the Big O.
$endgroup$
It may be helpful to understand that Big-O describes a set of functions. That is $O(f(n)) = lbrace g(n) | exists n,c>0: forall m > n: ctimes g(m) le f(m)rbrace$
The usage of $=$ is kind of unfortunate and using $in$ would make that relationship a lot clearer. but the set notation symbols are a bit difficult to type so now we are stuck with the current convention.
This then shows that $O(n) = O(2n)$ Or that constant factors don't matter when defining the Big O.
answered May 9 at 9:22
ratchet freakratchet freak
2,96399
2,96399
4
$begingroup$
The equality convention isn't really about typing. It's because the usefulness of expressions such as $log (n!) = nlog n - n + O(log n)$ encourages us to view $O(f)$ as both "the set of functions such that [...]" and "some function such that [...]"
$endgroup$
– David Richerby
May 9 at 9:26
add a comment |
4
$begingroup$
The equality convention isn't really about typing. It's because the usefulness of expressions such as $log (n!) = nlog n - n + O(log n)$ encourages us to view $O(f)$ as both "the set of functions such that [...]" and "some function such that [...]"
$endgroup$
– David Richerby
May 9 at 9:26
4
4
$begingroup$
The equality convention isn't really about typing. It's because the usefulness of expressions such as $log (n!) = nlog n - n + O(log n)$ encourages us to view $O(f)$ as both "the set of functions such that [...]" and "some function such that [...]"
$endgroup$
– David Richerby
May 9 at 9:26
$begingroup$
The equality convention isn't really about typing. It's because the usefulness of expressions such as $log (n!) = nlog n - n + O(log n)$ encourages us to view $O(f)$ as both "the set of functions such that [...]" and "some function such that [...]"
$endgroup$
– David Richerby
May 9 at 9:26
add a comment |
Thanks for contributing an answer to Computer Science Stack Exchange!
- Please be sure to answer the question. Provide details and share your research!
But avoid …
- Asking for help, clarification, or responding to other answers.
- Making statements based on opinion; back them up with references or personal experience.
Use MathJax to format equations. MathJax reference.
To learn more, see our tips on writing great answers.
Sign up or log in
StackExchange.ready(function ()
StackExchange.helpers.onClickDraftSave('#login-link');
);
Sign up using Google
Sign up using Facebook
Sign up using Email and Password
Post as a guest
Required, but never shown
StackExchange.ready(
function ()
StackExchange.openid.initPostLogin('.new-post-login', 'https%3a%2f%2fcs.stackexchange.com%2fquestions%2f109127%2fare-there-variations-of-the-regular-runtimes-of-the-big-o-notation%23new-answer', 'question_page');
);
Post as a guest
Required, but never shown
Sign up or log in
StackExchange.ready(function ()
StackExchange.helpers.onClickDraftSave('#login-link');
);
Sign up using Google
Sign up using Facebook
Sign up using Email and Password
Post as a guest
Required, but never shown
Sign up or log in
StackExchange.ready(function ()
StackExchange.helpers.onClickDraftSave('#login-link');
);
Sign up using Google
Sign up using Facebook
Sign up using Email and Password
Post as a guest
Required, but never shown
Sign up or log in
StackExchange.ready(function ()
StackExchange.helpers.onClickDraftSave('#login-link');
);
Sign up using Google
Sign up using Facebook
Sign up using Email and Password
Sign up using Google
Sign up using Facebook
Sign up using Email and Password
Post as a guest
Required, but never shown
Required, but never shown
Required, but never shown
Required, but never shown
Required, but never shown
Required, but never shown
Required, but never shown
Required, but never shown
Required, but never shown
Kq4y zVC T sfk,edcvEZ,OtY7SjoEJ7Tan5gnWQjsVGbNwAJFQXl,4t56lEoiaAlEReCRsT3l2i5K2HiVqgRU o C8HlVkphQ,x,7OfNFa11
$begingroup$
There is no material difference between O(5n^2) to a O(3n^2) during an asymptotic analysis. They are both O(n^2), and only differ by a constant. In fact, in a proof, you might even reduce O(5n^2) to O(3n^2) or O(n^2) to make the math cleaner since they are equivalent. When writing your proof, you make a note in a sidebar that they are equivalent. In fact, you might even swap a O(log n) with O(n) and note that O(log n) <= O(n) in the sidebar. The note in the sidebar tells the reader it is intentional, and not a typo. (At least that's how I did it when I took Algorithm Analysis in college).
$endgroup$
– jww
May 9 at 20:54
2
$begingroup$
If you're using the $O(cdot)$ notation to get rid of small factors, you can always write something like "... improves the running time from $5n^2 + o(n^2)$ down to $3n^2 + o(n^2)$", etc. Or, equivalently, $(5 + o(1))n^2$ and $(3 + o(1))n^2$. Some authors prefer to just write $sim 5n^2$ as shorthand for the former. See, for example, the textbook by Trefethen and Bau.
$endgroup$
– Yonatan N
May 10 at 0:14