Seasonality after 1st differencingWhen (and why) should you take the log of a distribution (of numbers)?Interpreting seasonality in ACF and PACF plotsTreating non-stationarity of time series in seasonal adjusted data with RTime Series: Seasonality and trendStationarity & seasonality in RContradiction in the ADF (Augmented Dickey-Fuller) and KPSS (Kwiatkowski–Phillips–Schmidt–Shin) tests for financial time seriesDoes seasonal differencing in SARIMA model take care of additive/ multiplicative seasonality?ACF indicates non-stationarity but but time series plot looks stationaryEffects of differencing on PACFHow to interpret autocorrelation plot?differencing in sARIMA modelsWhat values of ARIMA(p,d,q)(P,D,Q)[7] should I use?
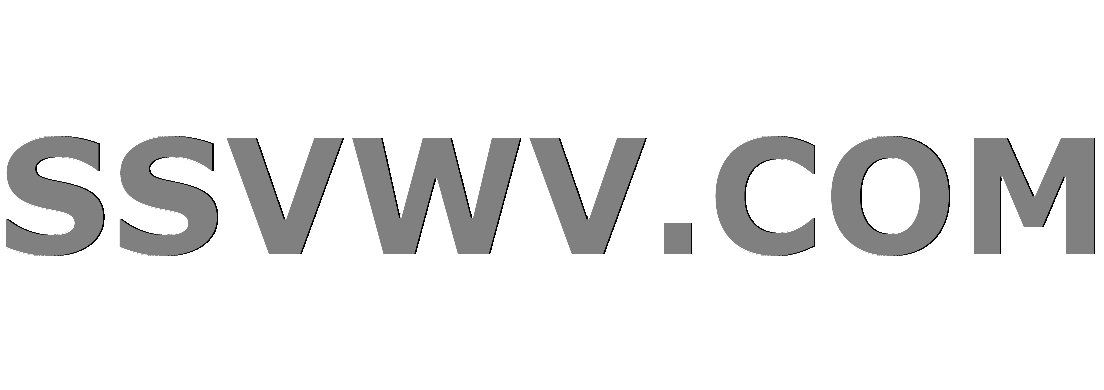
Multi tool use
Is adding a new player (or players) a DM decision, or a group decision?
“Faire” being used to mean “avoir l’air”?
How exactly is a normal force exerted, at the molecular level?
How hard is it to sell a home which is currently mortgaged?
If protons are the only stable baryons, why do they decay into neutrons in positron emission?
Averting Real Women Don’t Wear Dresses
can’t run a function against EXEC
When is it ok to add filler to a story?
Should I report a leak of confidential HR information?
What happens when your group is victim of a surprise attack but you can't be surprised?
Could Sauron have read Tom Bombadil's mind if Tom had held the Palantir?
The difference between Rad1 and Rfd1
Avoid bfseries from bolding pm in siunitx
Alphabet completion rate
If a high rpm motor is run at lower rpm, will it produce more torque?
How fast can a ship with rotating habitats be accelerated?
Should I hide continue button until tasks are completed?
How to write or read powers (math) by words?
How can I check type T is among parameter pack Ts... in C++?
Is there any set of 2-6 notes that doesn't have a chord name?
What shortcut does ⌦ symbol in Camunda macOS app indicate and how to invoke it?
Anagram Within an Anagram!
Symbol for "not absolutely continuous" in Latex
What are good ways to spray paint a QR code on a footpath?
Seasonality after 1st differencing
When (and why) should you take the log of a distribution (of numbers)?Interpreting seasonality in ACF and PACF plotsTreating non-stationarity of time series in seasonal adjusted data with RTime Series: Seasonality and trendStationarity & seasonality in RContradiction in the ADF (Augmented Dickey-Fuller) and KPSS (Kwiatkowski–Phillips–Schmidt–Shin) tests for financial time seriesDoes seasonal differencing in SARIMA model take care of additive/ multiplicative seasonality?ACF indicates non-stationarity but but time series plot looks stationaryEffects of differencing on PACFHow to interpret autocorrelation plot?differencing in sARIMA modelsWhat values of ARIMA(p,d,q)(P,D,Q)[7] should I use?
.everyoneloves__top-leaderboard:empty,.everyoneloves__mid-leaderboard:empty,.everyoneloves__bot-mid-leaderboard:empty margin-bottom:0;
$begingroup$
I am working with a financial time series (monthly frequency) and the raw data is not stationary according to ADF, KPSS. I then apply deflation (accounting for inflation), log transformation (to make an exponential trend linear) and lastly take the 1st differences. This series is not stationary.
When running the ACF/PACF on the first differences, I receive the following plot:
Which kind of suggests seasonality at 11 and 22 lags (This pattern was not visible before 1st differences). Does this imply I should apply another difference, now with lag 11 and potentially 22 to remove the seasonality?
EDIT: Thanks for the answers. The link to text data is here.
time-series stationarity seasonality acf-pacf
$endgroup$
add a comment |
$begingroup$
I am working with a financial time series (monthly frequency) and the raw data is not stationary according to ADF, KPSS. I then apply deflation (accounting for inflation), log transformation (to make an exponential trend linear) and lastly take the 1st differences. This series is not stationary.
When running the ACF/PACF on the first differences, I receive the following plot:
Which kind of suggests seasonality at 11 and 22 lags (This pattern was not visible before 1st differences). Does this imply I should apply another difference, now with lag 11 and potentially 22 to remove the seasonality?
EDIT: Thanks for the answers. The link to text data is here.
time-series stationarity seasonality acf-pacf
$endgroup$
add a comment |
$begingroup$
I am working with a financial time series (monthly frequency) and the raw data is not stationary according to ADF, KPSS. I then apply deflation (accounting for inflation), log transformation (to make an exponential trend linear) and lastly take the 1st differences. This series is not stationary.
When running the ACF/PACF on the first differences, I receive the following plot:
Which kind of suggests seasonality at 11 and 22 lags (This pattern was not visible before 1st differences). Does this imply I should apply another difference, now with lag 11 and potentially 22 to remove the seasonality?
EDIT: Thanks for the answers. The link to text data is here.
time-series stationarity seasonality acf-pacf
$endgroup$
I am working with a financial time series (monthly frequency) and the raw data is not stationary according to ADF, KPSS. I then apply deflation (accounting for inflation), log transformation (to make an exponential trend linear) and lastly take the 1st differences. This series is not stationary.
When running the ACF/PACF on the first differences, I receive the following plot:
Which kind of suggests seasonality at 11 and 22 lags (This pattern was not visible before 1st differences). Does this imply I should apply another difference, now with lag 11 and potentially 22 to remove the seasonality?
EDIT: Thanks for the answers. The link to text data is here.
time-series stationarity seasonality acf-pacf
time-series stationarity seasonality acf-pacf
edited Jun 9 at 21:03
abu
asked Jun 9 at 20:28
abuabu
819 bronze badges
819 bronze badges
add a comment |
add a comment |
2 Answers
2
active
oldest
votes
$begingroup$
The answer is no because you may have injected this phenomenon as a result of transforming the data in an unwarranted fashion ... see the Slutsky Effect where a linear (weighted ) combinations of i.i.d. values leads to a series with auto-correlative structure . Slutsky http://www-history.mcs.st-andrews.ac.uk/Biographies/Slutsky.html Effect ... Unnecesaary differencing can INJECT variability. Consider the variance of a random process that is differenced OR unnecessarily filtered http://mathworld.wolfram.com/Slutzky-YuleEffect.html
Non-stationarity is a symptom with possibly many causes. One cause is a shift in the mean at one or more points in time. Another possible cause is a change in parameters at one or more points in time. Another cause is a deterministic change in error variance at one or more points in time. Prof. Spyros Makridakis wrote an article http://www.insead.edu/facultyresearch/research/doc.cfm?did=46900 of the danger of using differencing to render a series stationary.
When (and why) should you take the log of a distribution (of numbers)? discusses when you should take a power transform i.e. to decouple the relationship between the Expected Value and the variance of the model's residuals.
You may be injecting structure via unwarranted transformations ( differencing is a transformation) .
Simply adjusting for a contemporaneous series (inflation) may be incorrect as the Y variable may be impacted by changes in the X variable or lags of the X variable.
This is why we build SARMAX models https://autobox.com/pdfs/SARMAX.pdf.
Why don't you post your original data in a csv format and I and others may
be able to help .
EDITED AFTER RECEIPT OF DATA:
I took your 132 monthly values into AUTOBOX ( a piece of software that I have helped to develop ) and automatically developed a useful model . It has a number of advanced features that can be helpful.
Here is the data which clearly suggests that as the series gets higher the variability increases. An even "truer" statement is that the variance changes at one point in time (around period 54) and not pervasively suggesting that a Weighted least Squares would be more appropriate than a Log Transform . This will be found via the TSAY test described here https://onlinelibrary.wiley.com/doi/abs/10.1002/for.3980070102 with an excerpt here
The TSAY test shown here led to a first difference model (nearly second differences as suggested by the ar coefficients nearly summing to 1.0 ) here
with 9 pulses/shocks and a positive level shift (intercept change) at period 68.
The model in more detail is here and here
The Actual , Fit and Forecast graph is here with MOnte-Carlo generated simulations leading to these forecasts and limits
The role of statistics is to separate the data into signal and noise thus the litmus test is "did the equation generate a suitable noise process" . I would say a loud "Yes" .
Here is the plot of the model's residuals with this acf
In summary a useful model requires that the data be treated for non-constant variance by employing Weighted Least Squares effectively discounting the values 54-132 . The arima model is (2,1,0)(0,0,0)12 with a constant and 1 level shift along with 9 pulses.
It can help to see a segment of the augmented data matrix with the pulses and level shift where the columns represent the latent deterministic structure that was "scraped" from the data .
Hope this helps you and the list better ( partially ) understand the extraction of signal from data. No seasonality is detected with the data given .
$endgroup$
$begingroup$
Thanks for a really detailed answer. I added a link to data.
$endgroup$
– abu
Jun 9 at 21:03
$begingroup$
Thank you for all the work and references! I will go over them in detail now.
$endgroup$
– abu
Jun 10 at 10:38
$begingroup$
"a Weighted least Squares would be more appropriate than a Log Transform" Does this mean you are using WLS to change multiplicative data into additive data? Do you have a reference for this?
$endgroup$
– Frank
Jun 10 at 23:04
$begingroup$
See onlinelibrary.wiley.com/doi/abs/10.1002/for.3980070102 and in particular this excerpt
$endgroup$
– IrishStat
Jun 11 at 1:44
$begingroup$
see stats.stackexchange.com/questions/412230/… for the excerpt where the w's are developed inversely related to the cahnge in variance . Portion of the data is multlplied by a constant ...the resultant data can then be modeled with an additive model
$endgroup$
– IrishStat
Jun 11 at 1:54
add a comment |
$begingroup$
The answer is no, there is no problem of seasonality and autocorrelation here.
ACF and PACF charts use mostly 95% confidence intervals. This means, that typically 5% of values happens to be outside this interval - even when process do not show any autocorrelation or partial autocorrelation. Such things just happen.
Also, seasonal series tend to have different ACF functions - they tend to have form of weaves as you can observe in this question.
$endgroup$
$begingroup$
These plots do exhibit precisely those wave patterns you mention, apparently contradicting your conclusion.
$endgroup$
– whuber♦
Jun 9 at 21:06
$begingroup$
I would not agree, that differentiated series differs significantly from the white noise, which also may be tested. ACF patterns for seasonal data should be much stronger. Besides - it is financial data. It rarely shows any patterns due to arbitration.
$endgroup$
– cure
Jun 9 at 21:18
$begingroup$
How much stronger would "much" stronger be? You seem to arguing in a circular fashion: because you don't expect the series to exhibit seasonality, you cannot agree that the PACF and ACF show evidence of seasonality!
$endgroup$
– whuber♦
Jun 9 at 21:21
$begingroup$
Significantly different from white noise.
$endgroup$
– cure
Jun 9 at 21:42
add a comment |
Your Answer
StackExchange.ready(function()
var channelOptions =
tags: "".split(" "),
id: "65"
;
initTagRenderer("".split(" "), "".split(" "), channelOptions);
StackExchange.using("externalEditor", function()
// Have to fire editor after snippets, if snippets enabled
if (StackExchange.settings.snippets.snippetsEnabled)
StackExchange.using("snippets", function()
createEditor();
);
else
createEditor();
);
function createEditor()
StackExchange.prepareEditor(
heartbeatType: 'answer',
autoActivateHeartbeat: false,
convertImagesToLinks: false,
noModals: true,
showLowRepImageUploadWarning: true,
reputationToPostImages: null,
bindNavPrevention: true,
postfix: "",
imageUploader:
brandingHtml: "Powered by u003ca class="icon-imgur-white" href="https://imgur.com/"u003eu003c/au003e",
contentPolicyHtml: "User contributions licensed under u003ca href="https://creativecommons.org/licenses/by-sa/3.0/"u003ecc by-sa 3.0 with attribution requiredu003c/au003e u003ca href="https://stackoverflow.com/legal/content-policy"u003e(content policy)u003c/au003e",
allowUrls: true
,
onDemand: true,
discardSelector: ".discard-answer"
,immediatelyShowMarkdownHelp:true
);
);
Sign up or log in
StackExchange.ready(function ()
StackExchange.helpers.onClickDraftSave('#login-link');
);
Sign up using Google
Sign up using Facebook
Sign up using Email and Password
Post as a guest
Required, but never shown
StackExchange.ready(
function ()
StackExchange.openid.initPostLogin('.new-post-login', 'https%3a%2f%2fstats.stackexchange.com%2fquestions%2f412230%2fseasonality-after-1st-differencing%23new-answer', 'question_page');
);
Post as a guest
Required, but never shown
2 Answers
2
active
oldest
votes
2 Answers
2
active
oldest
votes
active
oldest
votes
active
oldest
votes
$begingroup$
The answer is no because you may have injected this phenomenon as a result of transforming the data in an unwarranted fashion ... see the Slutsky Effect where a linear (weighted ) combinations of i.i.d. values leads to a series with auto-correlative structure . Slutsky http://www-history.mcs.st-andrews.ac.uk/Biographies/Slutsky.html Effect ... Unnecesaary differencing can INJECT variability. Consider the variance of a random process that is differenced OR unnecessarily filtered http://mathworld.wolfram.com/Slutzky-YuleEffect.html
Non-stationarity is a symptom with possibly many causes. One cause is a shift in the mean at one or more points in time. Another possible cause is a change in parameters at one or more points in time. Another cause is a deterministic change in error variance at one or more points in time. Prof. Spyros Makridakis wrote an article http://www.insead.edu/facultyresearch/research/doc.cfm?did=46900 of the danger of using differencing to render a series stationary.
When (and why) should you take the log of a distribution (of numbers)? discusses when you should take a power transform i.e. to decouple the relationship between the Expected Value and the variance of the model's residuals.
You may be injecting structure via unwarranted transformations ( differencing is a transformation) .
Simply adjusting for a contemporaneous series (inflation) may be incorrect as the Y variable may be impacted by changes in the X variable or lags of the X variable.
This is why we build SARMAX models https://autobox.com/pdfs/SARMAX.pdf.
Why don't you post your original data in a csv format and I and others may
be able to help .
EDITED AFTER RECEIPT OF DATA:
I took your 132 monthly values into AUTOBOX ( a piece of software that I have helped to develop ) and automatically developed a useful model . It has a number of advanced features that can be helpful.
Here is the data which clearly suggests that as the series gets higher the variability increases. An even "truer" statement is that the variance changes at one point in time (around period 54) and not pervasively suggesting that a Weighted least Squares would be more appropriate than a Log Transform . This will be found via the TSAY test described here https://onlinelibrary.wiley.com/doi/abs/10.1002/for.3980070102 with an excerpt here
The TSAY test shown here led to a first difference model (nearly second differences as suggested by the ar coefficients nearly summing to 1.0 ) here
with 9 pulses/shocks and a positive level shift (intercept change) at period 68.
The model in more detail is here and here
The Actual , Fit and Forecast graph is here with MOnte-Carlo generated simulations leading to these forecasts and limits
The role of statistics is to separate the data into signal and noise thus the litmus test is "did the equation generate a suitable noise process" . I would say a loud "Yes" .
Here is the plot of the model's residuals with this acf
In summary a useful model requires that the data be treated for non-constant variance by employing Weighted Least Squares effectively discounting the values 54-132 . The arima model is (2,1,0)(0,0,0)12 with a constant and 1 level shift along with 9 pulses.
It can help to see a segment of the augmented data matrix with the pulses and level shift where the columns represent the latent deterministic structure that was "scraped" from the data .
Hope this helps you and the list better ( partially ) understand the extraction of signal from data. No seasonality is detected with the data given .
$endgroup$
$begingroup$
Thanks for a really detailed answer. I added a link to data.
$endgroup$
– abu
Jun 9 at 21:03
$begingroup$
Thank you for all the work and references! I will go over them in detail now.
$endgroup$
– abu
Jun 10 at 10:38
$begingroup$
"a Weighted least Squares would be more appropriate than a Log Transform" Does this mean you are using WLS to change multiplicative data into additive data? Do you have a reference for this?
$endgroup$
– Frank
Jun 10 at 23:04
$begingroup$
See onlinelibrary.wiley.com/doi/abs/10.1002/for.3980070102 and in particular this excerpt
$endgroup$
– IrishStat
Jun 11 at 1:44
$begingroup$
see stats.stackexchange.com/questions/412230/… for the excerpt where the w's are developed inversely related to the cahnge in variance . Portion of the data is multlplied by a constant ...the resultant data can then be modeled with an additive model
$endgroup$
– IrishStat
Jun 11 at 1:54
add a comment |
$begingroup$
The answer is no because you may have injected this phenomenon as a result of transforming the data in an unwarranted fashion ... see the Slutsky Effect where a linear (weighted ) combinations of i.i.d. values leads to a series with auto-correlative structure . Slutsky http://www-history.mcs.st-andrews.ac.uk/Biographies/Slutsky.html Effect ... Unnecesaary differencing can INJECT variability. Consider the variance of a random process that is differenced OR unnecessarily filtered http://mathworld.wolfram.com/Slutzky-YuleEffect.html
Non-stationarity is a symptom with possibly many causes. One cause is a shift in the mean at one or more points in time. Another possible cause is a change in parameters at one or more points in time. Another cause is a deterministic change in error variance at one or more points in time. Prof. Spyros Makridakis wrote an article http://www.insead.edu/facultyresearch/research/doc.cfm?did=46900 of the danger of using differencing to render a series stationary.
When (and why) should you take the log of a distribution (of numbers)? discusses when you should take a power transform i.e. to decouple the relationship between the Expected Value and the variance of the model's residuals.
You may be injecting structure via unwarranted transformations ( differencing is a transformation) .
Simply adjusting for a contemporaneous series (inflation) may be incorrect as the Y variable may be impacted by changes in the X variable or lags of the X variable.
This is why we build SARMAX models https://autobox.com/pdfs/SARMAX.pdf.
Why don't you post your original data in a csv format and I and others may
be able to help .
EDITED AFTER RECEIPT OF DATA:
I took your 132 monthly values into AUTOBOX ( a piece of software that I have helped to develop ) and automatically developed a useful model . It has a number of advanced features that can be helpful.
Here is the data which clearly suggests that as the series gets higher the variability increases. An even "truer" statement is that the variance changes at one point in time (around period 54) and not pervasively suggesting that a Weighted least Squares would be more appropriate than a Log Transform . This will be found via the TSAY test described here https://onlinelibrary.wiley.com/doi/abs/10.1002/for.3980070102 with an excerpt here
The TSAY test shown here led to a first difference model (nearly second differences as suggested by the ar coefficients nearly summing to 1.0 ) here
with 9 pulses/shocks and a positive level shift (intercept change) at period 68.
The model in more detail is here and here
The Actual , Fit and Forecast graph is here with MOnte-Carlo generated simulations leading to these forecasts and limits
The role of statistics is to separate the data into signal and noise thus the litmus test is "did the equation generate a suitable noise process" . I would say a loud "Yes" .
Here is the plot of the model's residuals with this acf
In summary a useful model requires that the data be treated for non-constant variance by employing Weighted Least Squares effectively discounting the values 54-132 . The arima model is (2,1,0)(0,0,0)12 with a constant and 1 level shift along with 9 pulses.
It can help to see a segment of the augmented data matrix with the pulses and level shift where the columns represent the latent deterministic structure that was "scraped" from the data .
Hope this helps you and the list better ( partially ) understand the extraction of signal from data. No seasonality is detected with the data given .
$endgroup$
$begingroup$
Thanks for a really detailed answer. I added a link to data.
$endgroup$
– abu
Jun 9 at 21:03
$begingroup$
Thank you for all the work and references! I will go over them in detail now.
$endgroup$
– abu
Jun 10 at 10:38
$begingroup$
"a Weighted least Squares would be more appropriate than a Log Transform" Does this mean you are using WLS to change multiplicative data into additive data? Do you have a reference for this?
$endgroup$
– Frank
Jun 10 at 23:04
$begingroup$
See onlinelibrary.wiley.com/doi/abs/10.1002/for.3980070102 and in particular this excerpt
$endgroup$
– IrishStat
Jun 11 at 1:44
$begingroup$
see stats.stackexchange.com/questions/412230/… for the excerpt where the w's are developed inversely related to the cahnge in variance . Portion of the data is multlplied by a constant ...the resultant data can then be modeled with an additive model
$endgroup$
– IrishStat
Jun 11 at 1:54
add a comment |
$begingroup$
The answer is no because you may have injected this phenomenon as a result of transforming the data in an unwarranted fashion ... see the Slutsky Effect where a linear (weighted ) combinations of i.i.d. values leads to a series with auto-correlative structure . Slutsky http://www-history.mcs.st-andrews.ac.uk/Biographies/Slutsky.html Effect ... Unnecesaary differencing can INJECT variability. Consider the variance of a random process that is differenced OR unnecessarily filtered http://mathworld.wolfram.com/Slutzky-YuleEffect.html
Non-stationarity is a symptom with possibly many causes. One cause is a shift in the mean at one or more points in time. Another possible cause is a change in parameters at one or more points in time. Another cause is a deterministic change in error variance at one or more points in time. Prof. Spyros Makridakis wrote an article http://www.insead.edu/facultyresearch/research/doc.cfm?did=46900 of the danger of using differencing to render a series stationary.
When (and why) should you take the log of a distribution (of numbers)? discusses when you should take a power transform i.e. to decouple the relationship between the Expected Value and the variance of the model's residuals.
You may be injecting structure via unwarranted transformations ( differencing is a transformation) .
Simply adjusting for a contemporaneous series (inflation) may be incorrect as the Y variable may be impacted by changes in the X variable or lags of the X variable.
This is why we build SARMAX models https://autobox.com/pdfs/SARMAX.pdf.
Why don't you post your original data in a csv format and I and others may
be able to help .
EDITED AFTER RECEIPT OF DATA:
I took your 132 monthly values into AUTOBOX ( a piece of software that I have helped to develop ) and automatically developed a useful model . It has a number of advanced features that can be helpful.
Here is the data which clearly suggests that as the series gets higher the variability increases. An even "truer" statement is that the variance changes at one point in time (around period 54) and not pervasively suggesting that a Weighted least Squares would be more appropriate than a Log Transform . This will be found via the TSAY test described here https://onlinelibrary.wiley.com/doi/abs/10.1002/for.3980070102 with an excerpt here
The TSAY test shown here led to a first difference model (nearly second differences as suggested by the ar coefficients nearly summing to 1.0 ) here
with 9 pulses/shocks and a positive level shift (intercept change) at period 68.
The model in more detail is here and here
The Actual , Fit and Forecast graph is here with MOnte-Carlo generated simulations leading to these forecasts and limits
The role of statistics is to separate the data into signal and noise thus the litmus test is "did the equation generate a suitable noise process" . I would say a loud "Yes" .
Here is the plot of the model's residuals with this acf
In summary a useful model requires that the data be treated for non-constant variance by employing Weighted Least Squares effectively discounting the values 54-132 . The arima model is (2,1,0)(0,0,0)12 with a constant and 1 level shift along with 9 pulses.
It can help to see a segment of the augmented data matrix with the pulses and level shift where the columns represent the latent deterministic structure that was "scraped" from the data .
Hope this helps you and the list better ( partially ) understand the extraction of signal from data. No seasonality is detected with the data given .
$endgroup$
The answer is no because you may have injected this phenomenon as a result of transforming the data in an unwarranted fashion ... see the Slutsky Effect where a linear (weighted ) combinations of i.i.d. values leads to a series with auto-correlative structure . Slutsky http://www-history.mcs.st-andrews.ac.uk/Biographies/Slutsky.html Effect ... Unnecesaary differencing can INJECT variability. Consider the variance of a random process that is differenced OR unnecessarily filtered http://mathworld.wolfram.com/Slutzky-YuleEffect.html
Non-stationarity is a symptom with possibly many causes. One cause is a shift in the mean at one or more points in time. Another possible cause is a change in parameters at one or more points in time. Another cause is a deterministic change in error variance at one or more points in time. Prof. Spyros Makridakis wrote an article http://www.insead.edu/facultyresearch/research/doc.cfm?did=46900 of the danger of using differencing to render a series stationary.
When (and why) should you take the log of a distribution (of numbers)? discusses when you should take a power transform i.e. to decouple the relationship between the Expected Value and the variance of the model's residuals.
You may be injecting structure via unwarranted transformations ( differencing is a transformation) .
Simply adjusting for a contemporaneous series (inflation) may be incorrect as the Y variable may be impacted by changes in the X variable or lags of the X variable.
This is why we build SARMAX models https://autobox.com/pdfs/SARMAX.pdf.
Why don't you post your original data in a csv format and I and others may
be able to help .
EDITED AFTER RECEIPT OF DATA:
I took your 132 monthly values into AUTOBOX ( a piece of software that I have helped to develop ) and automatically developed a useful model . It has a number of advanced features that can be helpful.
Here is the data which clearly suggests that as the series gets higher the variability increases. An even "truer" statement is that the variance changes at one point in time (around period 54) and not pervasively suggesting that a Weighted least Squares would be more appropriate than a Log Transform . This will be found via the TSAY test described here https://onlinelibrary.wiley.com/doi/abs/10.1002/for.3980070102 with an excerpt here
The TSAY test shown here led to a first difference model (nearly second differences as suggested by the ar coefficients nearly summing to 1.0 ) here
with 9 pulses/shocks and a positive level shift (intercept change) at period 68.
The model in more detail is here and here
The Actual , Fit and Forecast graph is here with MOnte-Carlo generated simulations leading to these forecasts and limits
The role of statistics is to separate the data into signal and noise thus the litmus test is "did the equation generate a suitable noise process" . I would say a loud "Yes" .
Here is the plot of the model's residuals with this acf
In summary a useful model requires that the data be treated for non-constant variance by employing Weighted Least Squares effectively discounting the values 54-132 . The arima model is (2,1,0)(0,0,0)12 with a constant and 1 level shift along with 9 pulses.
It can help to see a segment of the augmented data matrix with the pulses and level shift where the columns represent the latent deterministic structure that was "scraped" from the data .
Hope this helps you and the list better ( partially ) understand the extraction of signal from data. No seasonality is detected with the data given .
edited Jun 9 at 22:30
answered Jun 9 at 20:40


IrishStatIrishStat
22.6k4 gold badges23 silver badges46 bronze badges
22.6k4 gold badges23 silver badges46 bronze badges
$begingroup$
Thanks for a really detailed answer. I added a link to data.
$endgroup$
– abu
Jun 9 at 21:03
$begingroup$
Thank you for all the work and references! I will go over them in detail now.
$endgroup$
– abu
Jun 10 at 10:38
$begingroup$
"a Weighted least Squares would be more appropriate than a Log Transform" Does this mean you are using WLS to change multiplicative data into additive data? Do you have a reference for this?
$endgroup$
– Frank
Jun 10 at 23:04
$begingroup$
See onlinelibrary.wiley.com/doi/abs/10.1002/for.3980070102 and in particular this excerpt
$endgroup$
– IrishStat
Jun 11 at 1:44
$begingroup$
see stats.stackexchange.com/questions/412230/… for the excerpt where the w's are developed inversely related to the cahnge in variance . Portion of the data is multlplied by a constant ...the resultant data can then be modeled with an additive model
$endgroup$
– IrishStat
Jun 11 at 1:54
add a comment |
$begingroup$
Thanks for a really detailed answer. I added a link to data.
$endgroup$
– abu
Jun 9 at 21:03
$begingroup$
Thank you for all the work and references! I will go over them in detail now.
$endgroup$
– abu
Jun 10 at 10:38
$begingroup$
"a Weighted least Squares would be more appropriate than a Log Transform" Does this mean you are using WLS to change multiplicative data into additive data? Do you have a reference for this?
$endgroup$
– Frank
Jun 10 at 23:04
$begingroup$
See onlinelibrary.wiley.com/doi/abs/10.1002/for.3980070102 and in particular this excerpt
$endgroup$
– IrishStat
Jun 11 at 1:44
$begingroup$
see stats.stackexchange.com/questions/412230/… for the excerpt where the w's are developed inversely related to the cahnge in variance . Portion of the data is multlplied by a constant ...the resultant data can then be modeled with an additive model
$endgroup$
– IrishStat
Jun 11 at 1:54
$begingroup$
Thanks for a really detailed answer. I added a link to data.
$endgroup$
– abu
Jun 9 at 21:03
$begingroup$
Thanks for a really detailed answer. I added a link to data.
$endgroup$
– abu
Jun 9 at 21:03
$begingroup$
Thank you for all the work and references! I will go over them in detail now.
$endgroup$
– abu
Jun 10 at 10:38
$begingroup$
Thank you for all the work and references! I will go over them in detail now.
$endgroup$
– abu
Jun 10 at 10:38
$begingroup$
"a Weighted least Squares would be more appropriate than a Log Transform" Does this mean you are using WLS to change multiplicative data into additive data? Do you have a reference for this?
$endgroup$
– Frank
Jun 10 at 23:04
$begingroup$
"a Weighted least Squares would be more appropriate than a Log Transform" Does this mean you are using WLS to change multiplicative data into additive data? Do you have a reference for this?
$endgroup$
– Frank
Jun 10 at 23:04
$begingroup$
See onlinelibrary.wiley.com/doi/abs/10.1002/for.3980070102 and in particular this excerpt
$endgroup$
– IrishStat
Jun 11 at 1:44
$begingroup$
See onlinelibrary.wiley.com/doi/abs/10.1002/for.3980070102 and in particular this excerpt
$endgroup$
– IrishStat
Jun 11 at 1:44
$begingroup$
see stats.stackexchange.com/questions/412230/… for the excerpt where the w's are developed inversely related to the cahnge in variance . Portion of the data is multlplied by a constant ...the resultant data can then be modeled with an additive model
$endgroup$
– IrishStat
Jun 11 at 1:54
$begingroup$
see stats.stackexchange.com/questions/412230/… for the excerpt where the w's are developed inversely related to the cahnge in variance . Portion of the data is multlplied by a constant ...the resultant data can then be modeled with an additive model
$endgroup$
– IrishStat
Jun 11 at 1:54
add a comment |
$begingroup$
The answer is no, there is no problem of seasonality and autocorrelation here.
ACF and PACF charts use mostly 95% confidence intervals. This means, that typically 5% of values happens to be outside this interval - even when process do not show any autocorrelation or partial autocorrelation. Such things just happen.
Also, seasonal series tend to have different ACF functions - they tend to have form of weaves as you can observe in this question.
$endgroup$
$begingroup$
These plots do exhibit precisely those wave patterns you mention, apparently contradicting your conclusion.
$endgroup$
– whuber♦
Jun 9 at 21:06
$begingroup$
I would not agree, that differentiated series differs significantly from the white noise, which also may be tested. ACF patterns for seasonal data should be much stronger. Besides - it is financial data. It rarely shows any patterns due to arbitration.
$endgroup$
– cure
Jun 9 at 21:18
$begingroup$
How much stronger would "much" stronger be? You seem to arguing in a circular fashion: because you don't expect the series to exhibit seasonality, you cannot agree that the PACF and ACF show evidence of seasonality!
$endgroup$
– whuber♦
Jun 9 at 21:21
$begingroup$
Significantly different from white noise.
$endgroup$
– cure
Jun 9 at 21:42
add a comment |
$begingroup$
The answer is no, there is no problem of seasonality and autocorrelation here.
ACF and PACF charts use mostly 95% confidence intervals. This means, that typically 5% of values happens to be outside this interval - even when process do not show any autocorrelation or partial autocorrelation. Such things just happen.
Also, seasonal series tend to have different ACF functions - they tend to have form of weaves as you can observe in this question.
$endgroup$
$begingroup$
These plots do exhibit precisely those wave patterns you mention, apparently contradicting your conclusion.
$endgroup$
– whuber♦
Jun 9 at 21:06
$begingroup$
I would not agree, that differentiated series differs significantly from the white noise, which also may be tested. ACF patterns for seasonal data should be much stronger. Besides - it is financial data. It rarely shows any patterns due to arbitration.
$endgroup$
– cure
Jun 9 at 21:18
$begingroup$
How much stronger would "much" stronger be? You seem to arguing in a circular fashion: because you don't expect the series to exhibit seasonality, you cannot agree that the PACF and ACF show evidence of seasonality!
$endgroup$
– whuber♦
Jun 9 at 21:21
$begingroup$
Significantly different from white noise.
$endgroup$
– cure
Jun 9 at 21:42
add a comment |
$begingroup$
The answer is no, there is no problem of seasonality and autocorrelation here.
ACF and PACF charts use mostly 95% confidence intervals. This means, that typically 5% of values happens to be outside this interval - even when process do not show any autocorrelation or partial autocorrelation. Such things just happen.
Also, seasonal series tend to have different ACF functions - they tend to have form of weaves as you can observe in this question.
$endgroup$
The answer is no, there is no problem of seasonality and autocorrelation here.
ACF and PACF charts use mostly 95% confidence intervals. This means, that typically 5% of values happens to be outside this interval - even when process do not show any autocorrelation or partial autocorrelation. Such things just happen.
Also, seasonal series tend to have different ACF functions - they tend to have form of weaves as you can observe in this question.
edited Jun 9 at 20:53
answered Jun 9 at 20:42
curecure
2411 silver badge7 bronze badges
2411 silver badge7 bronze badges
$begingroup$
These plots do exhibit precisely those wave patterns you mention, apparently contradicting your conclusion.
$endgroup$
– whuber♦
Jun 9 at 21:06
$begingroup$
I would not agree, that differentiated series differs significantly from the white noise, which also may be tested. ACF patterns for seasonal data should be much stronger. Besides - it is financial data. It rarely shows any patterns due to arbitration.
$endgroup$
– cure
Jun 9 at 21:18
$begingroup$
How much stronger would "much" stronger be? You seem to arguing in a circular fashion: because you don't expect the series to exhibit seasonality, you cannot agree that the PACF and ACF show evidence of seasonality!
$endgroup$
– whuber♦
Jun 9 at 21:21
$begingroup$
Significantly different from white noise.
$endgroup$
– cure
Jun 9 at 21:42
add a comment |
$begingroup$
These plots do exhibit precisely those wave patterns you mention, apparently contradicting your conclusion.
$endgroup$
– whuber♦
Jun 9 at 21:06
$begingroup$
I would not agree, that differentiated series differs significantly from the white noise, which also may be tested. ACF patterns for seasonal data should be much stronger. Besides - it is financial data. It rarely shows any patterns due to arbitration.
$endgroup$
– cure
Jun 9 at 21:18
$begingroup$
How much stronger would "much" stronger be? You seem to arguing in a circular fashion: because you don't expect the series to exhibit seasonality, you cannot agree that the PACF and ACF show evidence of seasonality!
$endgroup$
– whuber♦
Jun 9 at 21:21
$begingroup$
Significantly different from white noise.
$endgroup$
– cure
Jun 9 at 21:42
$begingroup$
These plots do exhibit precisely those wave patterns you mention, apparently contradicting your conclusion.
$endgroup$
– whuber♦
Jun 9 at 21:06
$begingroup$
These plots do exhibit precisely those wave patterns you mention, apparently contradicting your conclusion.
$endgroup$
– whuber♦
Jun 9 at 21:06
$begingroup$
I would not agree, that differentiated series differs significantly from the white noise, which also may be tested. ACF patterns for seasonal data should be much stronger. Besides - it is financial data. It rarely shows any patterns due to arbitration.
$endgroup$
– cure
Jun 9 at 21:18
$begingroup$
I would not agree, that differentiated series differs significantly from the white noise, which also may be tested. ACF patterns for seasonal data should be much stronger. Besides - it is financial data. It rarely shows any patterns due to arbitration.
$endgroup$
– cure
Jun 9 at 21:18
$begingroup$
How much stronger would "much" stronger be? You seem to arguing in a circular fashion: because you don't expect the series to exhibit seasonality, you cannot agree that the PACF and ACF show evidence of seasonality!
$endgroup$
– whuber♦
Jun 9 at 21:21
$begingroup$
How much stronger would "much" stronger be? You seem to arguing in a circular fashion: because you don't expect the series to exhibit seasonality, you cannot agree that the PACF and ACF show evidence of seasonality!
$endgroup$
– whuber♦
Jun 9 at 21:21
$begingroup$
Significantly different from white noise.
$endgroup$
– cure
Jun 9 at 21:42
$begingroup$
Significantly different from white noise.
$endgroup$
– cure
Jun 9 at 21:42
add a comment |
Thanks for contributing an answer to Cross Validated!
- Please be sure to answer the question. Provide details and share your research!
But avoid …
- Asking for help, clarification, or responding to other answers.
- Making statements based on opinion; back them up with references or personal experience.
Use MathJax to format equations. MathJax reference.
To learn more, see our tips on writing great answers.
Sign up or log in
StackExchange.ready(function ()
StackExchange.helpers.onClickDraftSave('#login-link');
);
Sign up using Google
Sign up using Facebook
Sign up using Email and Password
Post as a guest
Required, but never shown
StackExchange.ready(
function ()
StackExchange.openid.initPostLogin('.new-post-login', 'https%3a%2f%2fstats.stackexchange.com%2fquestions%2f412230%2fseasonality-after-1st-differencing%23new-answer', 'question_page');
);
Post as a guest
Required, but never shown
Sign up or log in
StackExchange.ready(function ()
StackExchange.helpers.onClickDraftSave('#login-link');
);
Sign up using Google
Sign up using Facebook
Sign up using Email and Password
Post as a guest
Required, but never shown
Sign up or log in
StackExchange.ready(function ()
StackExchange.helpers.onClickDraftSave('#login-link');
);
Sign up using Google
Sign up using Facebook
Sign up using Email and Password
Post as a guest
Required, but never shown
Sign up or log in
StackExchange.ready(function ()
StackExchange.helpers.onClickDraftSave('#login-link');
);
Sign up using Google
Sign up using Facebook
Sign up using Email and Password
Sign up using Google
Sign up using Facebook
Sign up using Email and Password
Post as a guest
Required, but never shown
Required, but never shown
Required, but never shown
Required, but never shown
Required, but never shown
Required, but never shown
Required, but never shown
Required, but never shown
Required, but never shown
7XecrbI,I,Kpz IGqVQpBmaBW,LIqwPN3QpC3mL3eKdn3bW0PUsZ nNCS0rD ti,CgX0ilwwyoC87U16 PRAn64u6jR 30 GWA1lMXuFcEyFP