Multiple regression results help Announcing the arrival of Valued Associate #679: Cesar Manara Planned maintenance scheduled April 23, 2019 at 23:30 UTC (7:30pm US/Eastern)Not-significant F but a significant coefficient in multiple linear regressionWhen to transform predictor variables when doing multiple regression?Combining multiple imputation results for hierarchical regression in SPSSHow to deal with different outcomes between pairwise correlations and multiple regressionProbing effects in a multivariate multiple regressionPredictor flipping sign in regression with no multicollinearityMeta analysis of Multiple regressionWhat is the difference between each predictor's standardized betas (from multiple regression) and it's Pearson's correlation coefficient?Multiple linear regression coefficients meaningWhat is the correct way to follow up a multivariate multiple regression?
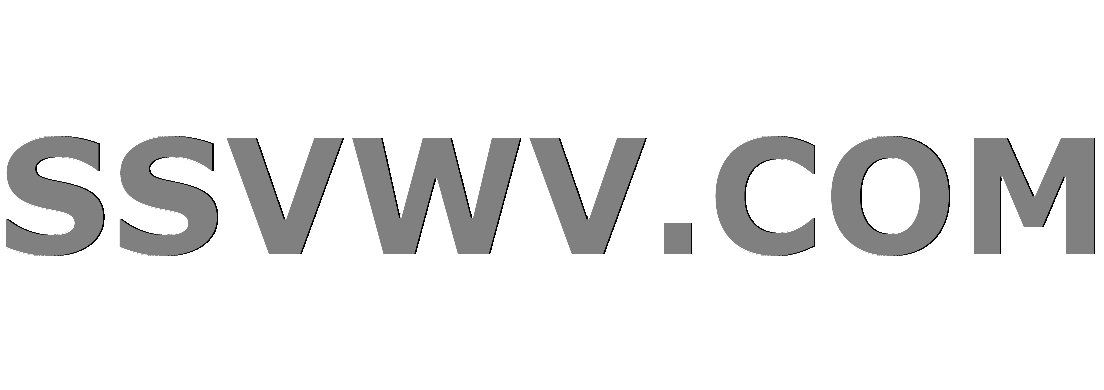
Multi tool use
Dyck paths with extra diagonals from valleys (Laser construction)
Do I really need to have a message in a novel to appeal to readers?
Does "shooting for effect" have contradictory meanings in different areas?
1-probability to calculate two events in a row
Crossing US/Canada Border for less than 24 hours
Google .dev domain strangely redirects to https
How do I find out the mythology and history of my Fortress?
How can I prevent/balance waiting and turtling as a response to cooldown mechanics
What is the difference between a "ranged attack" and a "ranged weapon attack"?
The test team as an enemy of development? And how can this be avoided?
Has negative voting ever been officially implemented in elections, or seriously proposed, or even studied?
How to report t statistic from R
How to write capital alpha?
One-one communication
How does the math work when buying airline miles?
How did Fremen produce and carry enough thumpers to use Sandworms as de facto Ubers?
Deconstruction is ambiguous
Misunderstanding of Sylow theory
A letter with no particular backstory
Is it possible for SQL statements to execute concurrently within a single session in SQL Server?
How often does castling occur in grandmaster games?
AppleTVs create a chatty alternate WiFi network
Converted a Scalar function to a TVF function for parallel execution-Still running in Serial mode
Can the Flaming Sphere spell be rammed into multiple Tiny creatures that are in the same 5-foot square?
Multiple regression results help
Announcing the arrival of Valued Associate #679: Cesar Manara
Planned maintenance scheduled April 23, 2019 at 23:30 UTC (7:30pm US/Eastern)Not-significant F but a significant coefficient in multiple linear regressionWhen to transform predictor variables when doing multiple regression?Combining multiple imputation results for hierarchical regression in SPSSHow to deal with different outcomes between pairwise correlations and multiple regressionProbing effects in a multivariate multiple regressionPredictor flipping sign in regression with no multicollinearityMeta analysis of Multiple regressionWhat is the difference between each predictor's standardized betas (from multiple regression) and it's Pearson's correlation coefficient?Multiple linear regression coefficients meaningWhat is the correct way to follow up a multivariate multiple regression?
.everyoneloves__top-leaderboard:empty,.everyoneloves__mid-leaderboard:empty,.everyoneloves__bot-mid-leaderboard:empty margin-bottom:0;
$begingroup$
For my first ever research paper I've run a hierarchal multiple linear regression with two predictors and one outcome variable, however I don't understand my results. I've found predictor A to be a significant predictor for my outcome variable alone. However, when both my predictors are in the model, predictor A is not a significant predictor, only predictor B is. How can this be if predictor A was significant in the first model? How does predictor B change how significant predictor A is?
Thank you!
multiple-regression mlr
New contributor
ummmm is a new contributor to this site. Take care in asking for clarification, commenting, and answering.
Check out our Code of Conduct.
$endgroup$
add a comment |
$begingroup$
For my first ever research paper I've run a hierarchal multiple linear regression with two predictors and one outcome variable, however I don't understand my results. I've found predictor A to be a significant predictor for my outcome variable alone. However, when both my predictors are in the model, predictor A is not a significant predictor, only predictor B is. How can this be if predictor A was significant in the first model? How does predictor B change how significant predictor A is?
Thank you!
multiple-regression mlr
New contributor
ummmm is a new contributor to this site. Take care in asking for clarification, commenting, and answering.
Check out our Code of Conduct.
$endgroup$
add a comment |
$begingroup$
For my first ever research paper I've run a hierarchal multiple linear regression with two predictors and one outcome variable, however I don't understand my results. I've found predictor A to be a significant predictor for my outcome variable alone. However, when both my predictors are in the model, predictor A is not a significant predictor, only predictor B is. How can this be if predictor A was significant in the first model? How does predictor B change how significant predictor A is?
Thank you!
multiple-regression mlr
New contributor
ummmm is a new contributor to this site. Take care in asking for clarification, commenting, and answering.
Check out our Code of Conduct.
$endgroup$
For my first ever research paper I've run a hierarchal multiple linear regression with two predictors and one outcome variable, however I don't understand my results. I've found predictor A to be a significant predictor for my outcome variable alone. However, when both my predictors are in the model, predictor A is not a significant predictor, only predictor B is. How can this be if predictor A was significant in the first model? How does predictor B change how significant predictor A is?
Thank you!
multiple-regression mlr
multiple-regression mlr
New contributor
ummmm is a new contributor to this site. Take care in asking for clarification, commenting, and answering.
Check out our Code of Conduct.
New contributor
ummmm is a new contributor to this site. Take care in asking for clarification, commenting, and answering.
Check out our Code of Conduct.
New contributor
ummmm is a new contributor to this site. Take care in asking for clarification, commenting, and answering.
Check out our Code of Conduct.
asked Apr 13 at 20:03
ummmmummmm
111
111
New contributor
ummmm is a new contributor to this site. Take care in asking for clarification, commenting, and answering.
Check out our Code of Conduct.
New contributor
ummmm is a new contributor to this site. Take care in asking for clarification, commenting, and answering.
Check out our Code of Conduct.
ummmm is a new contributor to this site. Take care in asking for clarification, commenting, and answering.
Check out our Code of Conduct.
add a comment |
add a comment |
3 Answers
3
active
oldest
votes
$begingroup$
regression coefficients reflect the simultaneous effects of multiple predictors. If the two predictors are inter-dependent (i.e. correlated) the results can differ from single input models.
$endgroup$
add a comment |
$begingroup$
The tests in multiple regression are "added last" tests. That means they test whether the model significantly improves after including the extra variable in a regression that contains all other predictors.
In your model with no predictors, adding A improves the model, so the test of A is significant in the model with only A.
In a model with A already in the model, adding B improves the model, so the test of B is significant in the model with A and B. But in a model with B already in the model, adding A doesn't improve the model, so the test of A is not significant in the model with A and B. B is doing all the work that A would do, so adding A doesn't improve the model beyond B.
As @IrishStat mentioned, this can occur when A and B are correlated (positively or negatively) with each other. It's a fairly common occurrence in regression modeling. The conclusion you might draw is that A predicts the outcome when B is not in the model (i.e., unavailable), but after including B, A doesn't do much more to predict the outcome. Unfortunately, without more information about the causal structure of your variables, there is little more interpretation available.
$endgroup$
add a comment |
$begingroup$
To expand a little on @Noah and @IrishStat's answers, in a multiple regression, coefficients for each independent variable/predictor are estimated to obtain the direct effect of each variable, using variation unique to that variable and the variable's correlation with the outcome variable, not using variation shared by predictors. (In technical terms, we are talking about variance and covariance of these variables.) The less unique variation there is, the less significant the estimate will become.
So why, in your example, did you end up with an insignificant predictor A when B was added, and not with a significant predictor A and insignificant predictor B? It is likely because the proportion of variance of predictor A that it has in common with predictor B is larger than the proportion of variance of predictor B that it has in common with predictor A.
$endgroup$
add a comment |
Your Answer
StackExchange.ready(function()
var channelOptions =
tags: "".split(" "),
id: "65"
;
initTagRenderer("".split(" "), "".split(" "), channelOptions);
StackExchange.using("externalEditor", function()
// Have to fire editor after snippets, if snippets enabled
if (StackExchange.settings.snippets.snippetsEnabled)
StackExchange.using("snippets", function()
createEditor();
);
else
createEditor();
);
function createEditor()
StackExchange.prepareEditor(
heartbeatType: 'answer',
autoActivateHeartbeat: false,
convertImagesToLinks: false,
noModals: true,
showLowRepImageUploadWarning: true,
reputationToPostImages: null,
bindNavPrevention: true,
postfix: "",
imageUploader:
brandingHtml: "Powered by u003ca class="icon-imgur-white" href="https://imgur.com/"u003eu003c/au003e",
contentPolicyHtml: "User contributions licensed under u003ca href="https://creativecommons.org/licenses/by-sa/3.0/"u003ecc by-sa 3.0 with attribution requiredu003c/au003e u003ca href="https://stackoverflow.com/legal/content-policy"u003e(content policy)u003c/au003e",
allowUrls: true
,
onDemand: true,
discardSelector: ".discard-answer"
,immediatelyShowMarkdownHelp:true
);
);
ummmm is a new contributor. Be nice, and check out our Code of Conduct.
Sign up or log in
StackExchange.ready(function ()
StackExchange.helpers.onClickDraftSave('#login-link');
);
Sign up using Google
Sign up using Facebook
Sign up using Email and Password
Post as a guest
Required, but never shown
StackExchange.ready(
function ()
StackExchange.openid.initPostLogin('.new-post-login', 'https%3a%2f%2fstats.stackexchange.com%2fquestions%2f402894%2fmultiple-regression-results-help%23new-answer', 'question_page');
);
Post as a guest
Required, but never shown
3 Answers
3
active
oldest
votes
3 Answers
3
active
oldest
votes
active
oldest
votes
active
oldest
votes
$begingroup$
regression coefficients reflect the simultaneous effects of multiple predictors. If the two predictors are inter-dependent (i.e. correlated) the results can differ from single input models.
$endgroup$
add a comment |
$begingroup$
regression coefficients reflect the simultaneous effects of multiple predictors. If the two predictors are inter-dependent (i.e. correlated) the results can differ from single input models.
$endgroup$
add a comment |
$begingroup$
regression coefficients reflect the simultaneous effects of multiple predictors. If the two predictors are inter-dependent (i.e. correlated) the results can differ from single input models.
$endgroup$
regression coefficients reflect the simultaneous effects of multiple predictors. If the two predictors are inter-dependent (i.e. correlated) the results can differ from single input models.
answered Apr 13 at 21:37


IrishStatIrishStat
21.6k42342
21.6k42342
add a comment |
add a comment |
$begingroup$
The tests in multiple regression are "added last" tests. That means they test whether the model significantly improves after including the extra variable in a regression that contains all other predictors.
In your model with no predictors, adding A improves the model, so the test of A is significant in the model with only A.
In a model with A already in the model, adding B improves the model, so the test of B is significant in the model with A and B. But in a model with B already in the model, adding A doesn't improve the model, so the test of A is not significant in the model with A and B. B is doing all the work that A would do, so adding A doesn't improve the model beyond B.
As @IrishStat mentioned, this can occur when A and B are correlated (positively or negatively) with each other. It's a fairly common occurrence in regression modeling. The conclusion you might draw is that A predicts the outcome when B is not in the model (i.e., unavailable), but after including B, A doesn't do much more to predict the outcome. Unfortunately, without more information about the causal structure of your variables, there is little more interpretation available.
$endgroup$
add a comment |
$begingroup$
The tests in multiple regression are "added last" tests. That means they test whether the model significantly improves after including the extra variable in a regression that contains all other predictors.
In your model with no predictors, adding A improves the model, so the test of A is significant in the model with only A.
In a model with A already in the model, adding B improves the model, so the test of B is significant in the model with A and B. But in a model with B already in the model, adding A doesn't improve the model, so the test of A is not significant in the model with A and B. B is doing all the work that A would do, so adding A doesn't improve the model beyond B.
As @IrishStat mentioned, this can occur when A and B are correlated (positively or negatively) with each other. It's a fairly common occurrence in regression modeling. The conclusion you might draw is that A predicts the outcome when B is not in the model (i.e., unavailable), but after including B, A doesn't do much more to predict the outcome. Unfortunately, without more information about the causal structure of your variables, there is little more interpretation available.
$endgroup$
add a comment |
$begingroup$
The tests in multiple regression are "added last" tests. That means they test whether the model significantly improves after including the extra variable in a regression that contains all other predictors.
In your model with no predictors, adding A improves the model, so the test of A is significant in the model with only A.
In a model with A already in the model, adding B improves the model, so the test of B is significant in the model with A and B. But in a model with B already in the model, adding A doesn't improve the model, so the test of A is not significant in the model with A and B. B is doing all the work that A would do, so adding A doesn't improve the model beyond B.
As @IrishStat mentioned, this can occur when A and B are correlated (positively or negatively) with each other. It's a fairly common occurrence in regression modeling. The conclusion you might draw is that A predicts the outcome when B is not in the model (i.e., unavailable), but after including B, A doesn't do much more to predict the outcome. Unfortunately, without more information about the causal structure of your variables, there is little more interpretation available.
$endgroup$
The tests in multiple regression are "added last" tests. That means they test whether the model significantly improves after including the extra variable in a regression that contains all other predictors.
In your model with no predictors, adding A improves the model, so the test of A is significant in the model with only A.
In a model with A already in the model, adding B improves the model, so the test of B is significant in the model with A and B. But in a model with B already in the model, adding A doesn't improve the model, so the test of A is not significant in the model with A and B. B is doing all the work that A would do, so adding A doesn't improve the model beyond B.
As @IrishStat mentioned, this can occur when A and B are correlated (positively or negatively) with each other. It's a fairly common occurrence in regression modeling. The conclusion you might draw is that A predicts the outcome when B is not in the model (i.e., unavailable), but after including B, A doesn't do much more to predict the outcome. Unfortunately, without more information about the causal structure of your variables, there is little more interpretation available.
answered Apr 13 at 22:29
NoahNoah
3,7461417
3,7461417
add a comment |
add a comment |
$begingroup$
To expand a little on @Noah and @IrishStat's answers, in a multiple regression, coefficients for each independent variable/predictor are estimated to obtain the direct effect of each variable, using variation unique to that variable and the variable's correlation with the outcome variable, not using variation shared by predictors. (In technical terms, we are talking about variance and covariance of these variables.) The less unique variation there is, the less significant the estimate will become.
So why, in your example, did you end up with an insignificant predictor A when B was added, and not with a significant predictor A and insignificant predictor B? It is likely because the proportion of variance of predictor A that it has in common with predictor B is larger than the proportion of variance of predictor B that it has in common with predictor A.
$endgroup$
add a comment |
$begingroup$
To expand a little on @Noah and @IrishStat's answers, in a multiple regression, coefficients for each independent variable/predictor are estimated to obtain the direct effect of each variable, using variation unique to that variable and the variable's correlation with the outcome variable, not using variation shared by predictors. (In technical terms, we are talking about variance and covariance of these variables.) The less unique variation there is, the less significant the estimate will become.
So why, in your example, did you end up with an insignificant predictor A when B was added, and not with a significant predictor A and insignificant predictor B? It is likely because the proportion of variance of predictor A that it has in common with predictor B is larger than the proportion of variance of predictor B that it has in common with predictor A.
$endgroup$
add a comment |
$begingroup$
To expand a little on @Noah and @IrishStat's answers, in a multiple regression, coefficients for each independent variable/predictor are estimated to obtain the direct effect of each variable, using variation unique to that variable and the variable's correlation with the outcome variable, not using variation shared by predictors. (In technical terms, we are talking about variance and covariance of these variables.) The less unique variation there is, the less significant the estimate will become.
So why, in your example, did you end up with an insignificant predictor A when B was added, and not with a significant predictor A and insignificant predictor B? It is likely because the proportion of variance of predictor A that it has in common with predictor B is larger than the proportion of variance of predictor B that it has in common with predictor A.
$endgroup$
To expand a little on @Noah and @IrishStat's answers, in a multiple regression, coefficients for each independent variable/predictor are estimated to obtain the direct effect of each variable, using variation unique to that variable and the variable's correlation with the outcome variable, not using variation shared by predictors. (In technical terms, we are talking about variance and covariance of these variables.) The less unique variation there is, the less significant the estimate will become.
So why, in your example, did you end up with an insignificant predictor A when B was added, and not with a significant predictor A and insignificant predictor B? It is likely because the proportion of variance of predictor A that it has in common with predictor B is larger than the proportion of variance of predictor B that it has in common with predictor A.
answered Apr 14 at 5:31


AlexKAlexK
2358
2358
add a comment |
add a comment |
ummmm is a new contributor. Be nice, and check out our Code of Conduct.
ummmm is a new contributor. Be nice, and check out our Code of Conduct.
ummmm is a new contributor. Be nice, and check out our Code of Conduct.
ummmm is a new contributor. Be nice, and check out our Code of Conduct.
Thanks for contributing an answer to Cross Validated!
- Please be sure to answer the question. Provide details and share your research!
But avoid …
- Asking for help, clarification, or responding to other answers.
- Making statements based on opinion; back them up with references or personal experience.
Use MathJax to format equations. MathJax reference.
To learn more, see our tips on writing great answers.
Sign up or log in
StackExchange.ready(function ()
StackExchange.helpers.onClickDraftSave('#login-link');
);
Sign up using Google
Sign up using Facebook
Sign up using Email and Password
Post as a guest
Required, but never shown
StackExchange.ready(
function ()
StackExchange.openid.initPostLogin('.new-post-login', 'https%3a%2f%2fstats.stackexchange.com%2fquestions%2f402894%2fmultiple-regression-results-help%23new-answer', 'question_page');
);
Post as a guest
Required, but never shown
Sign up or log in
StackExchange.ready(function ()
StackExchange.helpers.onClickDraftSave('#login-link');
);
Sign up using Google
Sign up using Facebook
Sign up using Email and Password
Post as a guest
Required, but never shown
Sign up or log in
StackExchange.ready(function ()
StackExchange.helpers.onClickDraftSave('#login-link');
);
Sign up using Google
Sign up using Facebook
Sign up using Email and Password
Post as a guest
Required, but never shown
Sign up or log in
StackExchange.ready(function ()
StackExchange.helpers.onClickDraftSave('#login-link');
);
Sign up using Google
Sign up using Facebook
Sign up using Email and Password
Sign up using Google
Sign up using Facebook
Sign up using Email and Password
Post as a guest
Required, but never shown
Required, but never shown
Required, but never shown
Required, but never shown
Required, but never shown
Required, but never shown
Required, but never shown
Required, but never shown
Required, but never shown
8Fku,m4xcr90SwJB0kEIpreI P,b,AQ,8MDi,ctWSnXl6INKvB yy