Pandas DataFrames: Create new rows with calculations across existing rows Announcing the arrival of Valued Associate #679: Cesar Manara Planned maintenance scheduled April 23, 2019 at 23:30 UTC (7:30pm US/Eastern) Data science time! April 2019 and salary with experience The Ask Question Wizard is Live!Dynamic Expression Evaluation in pandas using pd.eval()Add one row to pandas DataFrameSelecting multiple columns in a pandas dataframeAdding new column to existing DataFrame in Python pandasDelete column from pandas DataFrame by column nameHow to drop rows of Pandas DataFrame whose value in certain columns is NaN“Large data” work flows using pandasHow do I get the row count of a pandas DataFrame?How to iterate over rows in a DataFrame in Pandas?Select rows from a DataFrame based on values in a column in pandasGet list from pandas DataFrame column headers
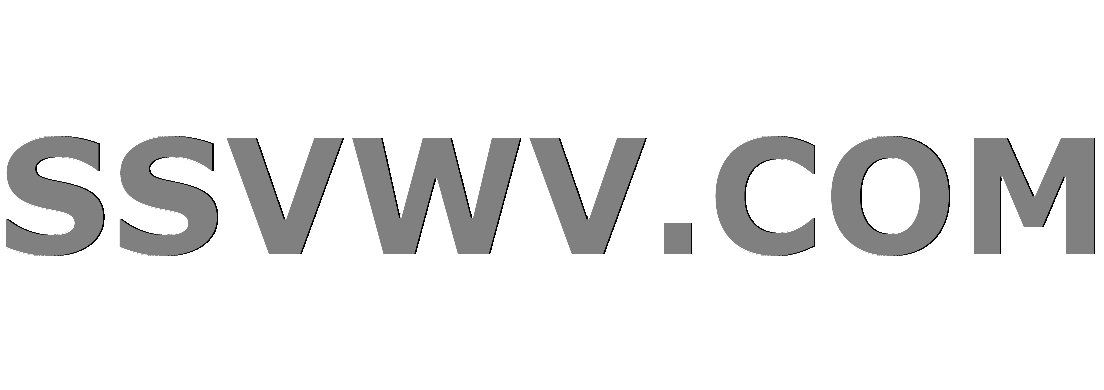
Multi tool use
Strange behavior of Object.defineProperty() in JavaScript
Can a sorcerer use careful spell on himself?
Can the Flaming Sphere spell be rammed into multiple Tiny creatures that are in the same 5-foot square?
Flash light on something
A letter with no particular backstory
Is it possible for SQL statements to execute concurrently within a single session in SQL Server?
Did any compiler fully use 80-bit floating point?
Why do early math courses focus on the cross sections of a cone and not on other 3D objects?
What to do with repeated rejections for phd position
What is the meaning of 'breadth' in breadth first search?
Is multiple magic items in one inherently imbalanced?
preposition before coffee
How to report t statistic from R
Karn the great creator - 'card from outside the game' in sealed
How does Belgium enforce obligatory attendance in elections?
What is the chair depicted in Cesare Maccari's 1889 painting "Cicerone denuncia Catilina"?
Most bit efficient text communication method?
How long can equipment go unused before powering up runs the risk of damage?
What are the discoveries that have been possible with the rejection of positivism?
Significance of Cersei's obsession with elephants?
Semigroups with no morphisms between them
Draw 4 of the same figure in the same tikzpicture
How would a mousetrap for use in space work?
How can I prevent/balance waiting and turtling as a response to cooldown mechanics
Pandas DataFrames: Create new rows with calculations across existing rows
Announcing the arrival of Valued Associate #679: Cesar Manara
Planned maintenance scheduled April 23, 2019 at 23:30 UTC (7:30pm US/Eastern)
Data science time! April 2019 and salary with experience
The Ask Question Wizard is Live!Dynamic Expression Evaluation in pandas using pd.eval()Add one row to pandas DataFrameSelecting multiple columns in a pandas dataframeAdding new column to existing DataFrame in Python pandasDelete column from pandas DataFrame by column nameHow to drop rows of Pandas DataFrame whose value in certain columns is NaN“Large data” work flows using pandasHow do I get the row count of a pandas DataFrame?How to iterate over rows in a DataFrame in Pandas?Select rows from a DataFrame based on values in a column in pandasGet list from pandas DataFrame column headers
.everyoneloves__top-leaderboard:empty,.everyoneloves__mid-leaderboard:empty,.everyoneloves__bot-mid-leaderboard:empty height:90px;width:728px;box-sizing:border-box;
How can I create new rows from an existing DataFrame by grouping by certain fields (in the example "Country" and "Industry") and applying some math to another field (in the example "Field" and "Value")?
Source DataFrame
df = pd.DataFrame('Country': ['USA','USA','USA','USA','USA','USA','Canada','Canada'],
'Industry': ['Finance', 'Finance', 'Retail',
'Retail', 'Energy', 'Energy',
'Retail', 'Retail'],
'Field': ['Import', 'Export','Import',
'Export','Import', 'Export',
'Import', 'Export'],
'Value': [100, 50, 80, 10, 20, 5, 30, 10])
Country Industry Field Value
0 USA Finance Import 100
1 USA Finance Export 50
2 USA Retail Import 80
3 USA Retail Export 10
4 USA Energy Import 20
5 USA Energy Export 5
6 Canada Retail Import 30
7 Canada Retail Export 10
Target DataFrame
Net = Import - Export
Country Industry Field Value
0 USA Finance Net 50
1 USA Retail Net 70
2 USA Energy Net 15
3 Canada Retail Net 20
python pandas dataframe
add a comment |
How can I create new rows from an existing DataFrame by grouping by certain fields (in the example "Country" and "Industry") and applying some math to another field (in the example "Field" and "Value")?
Source DataFrame
df = pd.DataFrame('Country': ['USA','USA','USA','USA','USA','USA','Canada','Canada'],
'Industry': ['Finance', 'Finance', 'Retail',
'Retail', 'Energy', 'Energy',
'Retail', 'Retail'],
'Field': ['Import', 'Export','Import',
'Export','Import', 'Export',
'Import', 'Export'],
'Value': [100, 50, 80, 10, 20, 5, 30, 10])
Country Industry Field Value
0 USA Finance Import 100
1 USA Finance Export 50
2 USA Retail Import 80
3 USA Retail Export 10
4 USA Energy Import 20
5 USA Energy Export 5
6 Canada Retail Import 30
7 Canada Retail Export 10
Target DataFrame
Net = Import - Export
Country Industry Field Value
0 USA Finance Net 50
1 USA Retail Net 70
2 USA Energy Net 15
3 Canada Retail Net 20
python pandas dataframe
add a comment |
How can I create new rows from an existing DataFrame by grouping by certain fields (in the example "Country" and "Industry") and applying some math to another field (in the example "Field" and "Value")?
Source DataFrame
df = pd.DataFrame('Country': ['USA','USA','USA','USA','USA','USA','Canada','Canada'],
'Industry': ['Finance', 'Finance', 'Retail',
'Retail', 'Energy', 'Energy',
'Retail', 'Retail'],
'Field': ['Import', 'Export','Import',
'Export','Import', 'Export',
'Import', 'Export'],
'Value': [100, 50, 80, 10, 20, 5, 30, 10])
Country Industry Field Value
0 USA Finance Import 100
1 USA Finance Export 50
2 USA Retail Import 80
3 USA Retail Export 10
4 USA Energy Import 20
5 USA Energy Export 5
6 Canada Retail Import 30
7 Canada Retail Export 10
Target DataFrame
Net = Import - Export
Country Industry Field Value
0 USA Finance Net 50
1 USA Retail Net 70
2 USA Energy Net 15
3 Canada Retail Net 20
python pandas dataframe
How can I create new rows from an existing DataFrame by grouping by certain fields (in the example "Country" and "Industry") and applying some math to another field (in the example "Field" and "Value")?
Source DataFrame
df = pd.DataFrame('Country': ['USA','USA','USA','USA','USA','USA','Canada','Canada'],
'Industry': ['Finance', 'Finance', 'Retail',
'Retail', 'Energy', 'Energy',
'Retail', 'Retail'],
'Field': ['Import', 'Export','Import',
'Export','Import', 'Export',
'Import', 'Export'],
'Value': [100, 50, 80, 10, 20, 5, 30, 10])
Country Industry Field Value
0 USA Finance Import 100
1 USA Finance Export 50
2 USA Retail Import 80
3 USA Retail Export 10
4 USA Energy Import 20
5 USA Energy Export 5
6 Canada Retail Import 30
7 Canada Retail Export 10
Target DataFrame
Net = Import - Export
Country Industry Field Value
0 USA Finance Net 50
1 USA Retail Net 70
2 USA Energy Net 15
3 Canada Retail Net 20
python pandas dataframe
python pandas dataframe
edited Apr 13 at 22:45


Scott Boston
58.9k73258
58.9k73258
asked Apr 13 at 21:53


LorenzLorenz
746
746
add a comment |
add a comment |
5 Answers
5
active
oldest
votes
There are quite possibly many ways. Here's one using groupby
and unstack
:
(df.groupby(['Country', 'Industry', 'Field'], sort=False)['Value']
.sum()
.unstack('Field')
.eval('Import - Export')
.reset_index(name='Value'))
Country Industry Value
0 USA Finance 50
1 USA Retail 70
2 USA Energy 15
3 Canada Retail 20
1
By far the best answer. Theunstack
followed byeval
is a really nice trick — better than a secondgroupby
andget_group
I would have done
– BallpointBen
Apr 13 at 22:17
1
@BallpointBeneval
andquery
are personal favourites of mine from the API. I've made attempts to popularise their use, but their usage is not completely understood. I have a QnA here, if you are interested.
– cs95
Apr 13 at 22:19
Works like a charm. Thank you very much. Very small comment - there is a closing bracket missing in the last line.
– Lorenz
Apr 14 at 1:40
@Lorenz Oops... fixed, thanks!
– cs95
Apr 14 at 1:41
@coldspeed Actually I think there’s a better way… see my answer.unstack
is expensive because it reshapes. Using the structure of the first groupby is more efficient, although it takes two lines.
– BallpointBen
Apr 14 at 3:03
|
show 1 more comment
IIUC
df=df.set_index(['Country','Industry'])
Newdf=(df.loc[df.Field=='Export','Value']-df.loc[df.Field=='Import','Value']).reset_index().assign(Field='Net')
Newdf
Country Industry Value Field
0 USA Finance -50 Net
1 USA Retail -70 Net
2 USA Energy -15 Net
3 Canada Retail -20 Net
pivot_table
df.pivot_table(index=['Country','Industry'],columns='Field',values='Value',aggfunc='sum').
diff(axis=1).
dropna(1).
rename(columns='Import':'Value').
reset_index()
Out[112]:
Field Country Industry Value
0 Canada Retail 20.0
1 USA Energy 15.0
2 USA Finance 50.0
3 USA Retail 70.0
add a comment |
You can use Groupby.diff()
and after that recreate the Field
column and finally use DataFrame.dropna
:
df['Value'] = df.groupby(['Country', 'Industry'])['Value'].diff().abs()
df['Field'] = 'Net'
df.dropna(inplace=True)
df.reset_index(drop=True, inplace=True)
print(df)
Country Industry Field Value
0 USA Finance Net 50.0
1 USA Retail Net 70.0
2 USA Energy Net 15.0
3 Canada Retail Net 20.0
add a comment |
You can do it this way to add those rows to your original dataframe:
df.set_index(['Country','Industry','Field'])
.unstack()['Value']
.eval('Net = Import - Export')
.stack().rename('Value').reset_index()
Output:
Country Industry Field Value
0 Canada Retail Export 10
1 Canada Retail Import 30
2 Canada Retail Net 20
3 USA Energy Export 5
4 USA Energy Import 20
5 USA Energy Net 15
6 USA Finance Export 50
7 USA Finance Import 100
8 USA Finance Net 50
9 USA Retail Export 10
10 USA Retail Import 80
11 USA Retail Net 70
Thanks - actually, I wanted to append it to the original df. So, nice trick to do this all in one command,
– Lorenz
Apr 14 at 1:41
1
Coldspeed‘s answer was a slight better fit to my overall code. Took from your code how you appended the result to the original df. Very tight result, though. Pitty that i can not accept two answers. But thanks again!
– Lorenz
Apr 14 at 3:07
add a comment |
This answer takes advantage of the fact that pandas puts the group keys in the multiindex of the resulting dataframe. (If there were only one group key, you could use loc
.)
>>> s = df.groupby(['Country', 'Industry', 'Field'])['Value'].sum()
>>> s.xs('Import', axis=0, level='Field') - s.xs('Export', axis=0, level='Field')
Country Industry
Canada Retail 20
USA Energy 15
Finance 50
Retail 70
Name: Value, dtype: int64
add a comment |
Your Answer
StackExchange.ifUsing("editor", function ()
StackExchange.using("externalEditor", function ()
StackExchange.using("snippets", function ()
StackExchange.snippets.init();
);
);
, "code-snippets");
StackExchange.ready(function()
var channelOptions =
tags: "".split(" "),
id: "1"
;
initTagRenderer("".split(" "), "".split(" "), channelOptions);
StackExchange.using("externalEditor", function()
// Have to fire editor after snippets, if snippets enabled
if (StackExchange.settings.snippets.snippetsEnabled)
StackExchange.using("snippets", function()
createEditor();
);
else
createEditor();
);
function createEditor()
StackExchange.prepareEditor(
heartbeatType: 'answer',
autoActivateHeartbeat: false,
convertImagesToLinks: true,
noModals: true,
showLowRepImageUploadWarning: true,
reputationToPostImages: 10,
bindNavPrevention: true,
postfix: "",
imageUploader:
brandingHtml: "Powered by u003ca class="icon-imgur-white" href="https://imgur.com/"u003eu003c/au003e",
contentPolicyHtml: "User contributions licensed under u003ca href="https://creativecommons.org/licenses/by-sa/3.0/"u003ecc by-sa 3.0 with attribution requiredu003c/au003e u003ca href="https://stackoverflow.com/legal/content-policy"u003e(content policy)u003c/au003e",
allowUrls: true
,
onDemand: true,
discardSelector: ".discard-answer"
,immediatelyShowMarkdownHelp:true
);
);
Sign up or log in
StackExchange.ready(function ()
StackExchange.helpers.onClickDraftSave('#login-link');
);
Sign up using Google
Sign up using Facebook
Sign up using Email and Password
Post as a guest
Required, but never shown
StackExchange.ready(
function ()
StackExchange.openid.initPostLogin('.new-post-login', 'https%3a%2f%2fstackoverflow.com%2fquestions%2f55670192%2fpandas-dataframes-create-new-rows-with-calculations-across-existing-rows%23new-answer', 'question_page');
);
Post as a guest
Required, but never shown
5 Answers
5
active
oldest
votes
5 Answers
5
active
oldest
votes
active
oldest
votes
active
oldest
votes
There are quite possibly many ways. Here's one using groupby
and unstack
:
(df.groupby(['Country', 'Industry', 'Field'], sort=False)['Value']
.sum()
.unstack('Field')
.eval('Import - Export')
.reset_index(name='Value'))
Country Industry Value
0 USA Finance 50
1 USA Retail 70
2 USA Energy 15
3 Canada Retail 20
1
By far the best answer. Theunstack
followed byeval
is a really nice trick — better than a secondgroupby
andget_group
I would have done
– BallpointBen
Apr 13 at 22:17
1
@BallpointBeneval
andquery
are personal favourites of mine from the API. I've made attempts to popularise their use, but their usage is not completely understood. I have a QnA here, if you are interested.
– cs95
Apr 13 at 22:19
Works like a charm. Thank you very much. Very small comment - there is a closing bracket missing in the last line.
– Lorenz
Apr 14 at 1:40
@Lorenz Oops... fixed, thanks!
– cs95
Apr 14 at 1:41
@coldspeed Actually I think there’s a better way… see my answer.unstack
is expensive because it reshapes. Using the structure of the first groupby is more efficient, although it takes two lines.
– BallpointBen
Apr 14 at 3:03
|
show 1 more comment
There are quite possibly many ways. Here's one using groupby
and unstack
:
(df.groupby(['Country', 'Industry', 'Field'], sort=False)['Value']
.sum()
.unstack('Field')
.eval('Import - Export')
.reset_index(name='Value'))
Country Industry Value
0 USA Finance 50
1 USA Retail 70
2 USA Energy 15
3 Canada Retail 20
1
By far the best answer. Theunstack
followed byeval
is a really nice trick — better than a secondgroupby
andget_group
I would have done
– BallpointBen
Apr 13 at 22:17
1
@BallpointBeneval
andquery
are personal favourites of mine from the API. I've made attempts to popularise their use, but their usage is not completely understood. I have a QnA here, if you are interested.
– cs95
Apr 13 at 22:19
Works like a charm. Thank you very much. Very small comment - there is a closing bracket missing in the last line.
– Lorenz
Apr 14 at 1:40
@Lorenz Oops... fixed, thanks!
– cs95
Apr 14 at 1:41
@coldspeed Actually I think there’s a better way… see my answer.unstack
is expensive because it reshapes. Using the structure of the first groupby is more efficient, although it takes two lines.
– BallpointBen
Apr 14 at 3:03
|
show 1 more comment
There are quite possibly many ways. Here's one using groupby
and unstack
:
(df.groupby(['Country', 'Industry', 'Field'], sort=False)['Value']
.sum()
.unstack('Field')
.eval('Import - Export')
.reset_index(name='Value'))
Country Industry Value
0 USA Finance 50
1 USA Retail 70
2 USA Energy 15
3 Canada Retail 20
There are quite possibly many ways. Here's one using groupby
and unstack
:
(df.groupby(['Country', 'Industry', 'Field'], sort=False)['Value']
.sum()
.unstack('Field')
.eval('Import - Export')
.reset_index(name='Value'))
Country Industry Value
0 USA Finance 50
1 USA Retail 70
2 USA Energy 15
3 Canada Retail 20
edited Apr 14 at 1:41
answered Apr 13 at 21:56


cs95cs95
143k25164249
143k25164249
1
By far the best answer. Theunstack
followed byeval
is a really nice trick — better than a secondgroupby
andget_group
I would have done
– BallpointBen
Apr 13 at 22:17
1
@BallpointBeneval
andquery
are personal favourites of mine from the API. I've made attempts to popularise their use, but their usage is not completely understood. I have a QnA here, if you are interested.
– cs95
Apr 13 at 22:19
Works like a charm. Thank you very much. Very small comment - there is a closing bracket missing in the last line.
– Lorenz
Apr 14 at 1:40
@Lorenz Oops... fixed, thanks!
– cs95
Apr 14 at 1:41
@coldspeed Actually I think there’s a better way… see my answer.unstack
is expensive because it reshapes. Using the structure of the first groupby is more efficient, although it takes two lines.
– BallpointBen
Apr 14 at 3:03
|
show 1 more comment
1
By far the best answer. Theunstack
followed byeval
is a really nice trick — better than a secondgroupby
andget_group
I would have done
– BallpointBen
Apr 13 at 22:17
1
@BallpointBeneval
andquery
are personal favourites of mine from the API. I've made attempts to popularise their use, but their usage is not completely understood. I have a QnA here, if you are interested.
– cs95
Apr 13 at 22:19
Works like a charm. Thank you very much. Very small comment - there is a closing bracket missing in the last line.
– Lorenz
Apr 14 at 1:40
@Lorenz Oops... fixed, thanks!
– cs95
Apr 14 at 1:41
@coldspeed Actually I think there’s a better way… see my answer.unstack
is expensive because it reshapes. Using the structure of the first groupby is more efficient, although it takes two lines.
– BallpointBen
Apr 14 at 3:03
1
1
By far the best answer. The
unstack
followed by eval
is a really nice trick — better than a second groupby
and get_group
I would have done– BallpointBen
Apr 13 at 22:17
By far the best answer. The
unstack
followed by eval
is a really nice trick — better than a second groupby
and get_group
I would have done– BallpointBen
Apr 13 at 22:17
1
1
@BallpointBen
eval
and query
are personal favourites of mine from the API. I've made attempts to popularise their use, but their usage is not completely understood. I have a QnA here, if you are interested.– cs95
Apr 13 at 22:19
@BallpointBen
eval
and query
are personal favourites of mine from the API. I've made attempts to popularise their use, but their usage is not completely understood. I have a QnA here, if you are interested.– cs95
Apr 13 at 22:19
Works like a charm. Thank you very much. Very small comment - there is a closing bracket missing in the last line.
– Lorenz
Apr 14 at 1:40
Works like a charm. Thank you very much. Very small comment - there is a closing bracket missing in the last line.
– Lorenz
Apr 14 at 1:40
@Lorenz Oops... fixed, thanks!
– cs95
Apr 14 at 1:41
@Lorenz Oops... fixed, thanks!
– cs95
Apr 14 at 1:41
@coldspeed Actually I think there’s a better way… see my answer.
unstack
is expensive because it reshapes. Using the structure of the first groupby is more efficient, although it takes two lines.– BallpointBen
Apr 14 at 3:03
@coldspeed Actually I think there’s a better way… see my answer.
unstack
is expensive because it reshapes. Using the structure of the first groupby is more efficient, although it takes two lines.– BallpointBen
Apr 14 at 3:03
|
show 1 more comment
IIUC
df=df.set_index(['Country','Industry'])
Newdf=(df.loc[df.Field=='Export','Value']-df.loc[df.Field=='Import','Value']).reset_index().assign(Field='Net')
Newdf
Country Industry Value Field
0 USA Finance -50 Net
1 USA Retail -70 Net
2 USA Energy -15 Net
3 Canada Retail -20 Net
pivot_table
df.pivot_table(index=['Country','Industry'],columns='Field',values='Value',aggfunc='sum').
diff(axis=1).
dropna(1).
rename(columns='Import':'Value').
reset_index()
Out[112]:
Field Country Industry Value
0 Canada Retail 20.0
1 USA Energy 15.0
2 USA Finance 50.0
3 USA Retail 70.0
add a comment |
IIUC
df=df.set_index(['Country','Industry'])
Newdf=(df.loc[df.Field=='Export','Value']-df.loc[df.Field=='Import','Value']).reset_index().assign(Field='Net')
Newdf
Country Industry Value Field
0 USA Finance -50 Net
1 USA Retail -70 Net
2 USA Energy -15 Net
3 Canada Retail -20 Net
pivot_table
df.pivot_table(index=['Country','Industry'],columns='Field',values='Value',aggfunc='sum').
diff(axis=1).
dropna(1).
rename(columns='Import':'Value').
reset_index()
Out[112]:
Field Country Industry Value
0 Canada Retail 20.0
1 USA Energy 15.0
2 USA Finance 50.0
3 USA Retail 70.0
add a comment |
IIUC
df=df.set_index(['Country','Industry'])
Newdf=(df.loc[df.Field=='Export','Value']-df.loc[df.Field=='Import','Value']).reset_index().assign(Field='Net')
Newdf
Country Industry Value Field
0 USA Finance -50 Net
1 USA Retail -70 Net
2 USA Energy -15 Net
3 Canada Retail -20 Net
pivot_table
df.pivot_table(index=['Country','Industry'],columns='Field',values='Value',aggfunc='sum').
diff(axis=1).
dropna(1).
rename(columns='Import':'Value').
reset_index()
Out[112]:
Field Country Industry Value
0 Canada Retail 20.0
1 USA Energy 15.0
2 USA Finance 50.0
3 USA Retail 70.0
IIUC
df=df.set_index(['Country','Industry'])
Newdf=(df.loc[df.Field=='Export','Value']-df.loc[df.Field=='Import','Value']).reset_index().assign(Field='Net')
Newdf
Country Industry Value Field
0 USA Finance -50 Net
1 USA Retail -70 Net
2 USA Energy -15 Net
3 Canada Retail -20 Net
pivot_table
df.pivot_table(index=['Country','Industry'],columns='Field',values='Value',aggfunc='sum').
diff(axis=1).
dropna(1).
rename(columns='Import':'Value').
reset_index()
Out[112]:
Field Country Industry Value
0 Canada Retail 20.0
1 USA Energy 15.0
2 USA Finance 50.0
3 USA Retail 70.0
edited Apr 13 at 23:12
answered Apr 13 at 21:58


Wen-BenWen-Ben
127k83872
127k83872
add a comment |
add a comment |
You can use Groupby.diff()
and after that recreate the Field
column and finally use DataFrame.dropna
:
df['Value'] = df.groupby(['Country', 'Industry'])['Value'].diff().abs()
df['Field'] = 'Net'
df.dropna(inplace=True)
df.reset_index(drop=True, inplace=True)
print(df)
Country Industry Field Value
0 USA Finance Net 50.0
1 USA Retail Net 70.0
2 USA Energy Net 15.0
3 Canada Retail Net 20.0
add a comment |
You can use Groupby.diff()
and after that recreate the Field
column and finally use DataFrame.dropna
:
df['Value'] = df.groupby(['Country', 'Industry'])['Value'].diff().abs()
df['Field'] = 'Net'
df.dropna(inplace=True)
df.reset_index(drop=True, inplace=True)
print(df)
Country Industry Field Value
0 USA Finance Net 50.0
1 USA Retail Net 70.0
2 USA Energy Net 15.0
3 Canada Retail Net 20.0
add a comment |
You can use Groupby.diff()
and after that recreate the Field
column and finally use DataFrame.dropna
:
df['Value'] = df.groupby(['Country', 'Industry'])['Value'].diff().abs()
df['Field'] = 'Net'
df.dropna(inplace=True)
df.reset_index(drop=True, inplace=True)
print(df)
Country Industry Field Value
0 USA Finance Net 50.0
1 USA Retail Net 70.0
2 USA Energy Net 15.0
3 Canada Retail Net 20.0
You can use Groupby.diff()
and after that recreate the Field
column and finally use DataFrame.dropna
:
df['Value'] = df.groupby(['Country', 'Industry'])['Value'].diff().abs()
df['Field'] = 'Net'
df.dropna(inplace=True)
df.reset_index(drop=True, inplace=True)
print(df)
Country Industry Field Value
0 USA Finance Net 50.0
1 USA Retail Net 70.0
2 USA Energy Net 15.0
3 Canada Retail Net 20.0
answered Apr 13 at 22:05


ErfanErfan
3,6961419
3,6961419
add a comment |
add a comment |
You can do it this way to add those rows to your original dataframe:
df.set_index(['Country','Industry','Field'])
.unstack()['Value']
.eval('Net = Import - Export')
.stack().rename('Value').reset_index()
Output:
Country Industry Field Value
0 Canada Retail Export 10
1 Canada Retail Import 30
2 Canada Retail Net 20
3 USA Energy Export 5
4 USA Energy Import 20
5 USA Energy Net 15
6 USA Finance Export 50
7 USA Finance Import 100
8 USA Finance Net 50
9 USA Retail Export 10
10 USA Retail Import 80
11 USA Retail Net 70
Thanks - actually, I wanted to append it to the original df. So, nice trick to do this all in one command,
– Lorenz
Apr 14 at 1:41
1
Coldspeed‘s answer was a slight better fit to my overall code. Took from your code how you appended the result to the original df. Very tight result, though. Pitty that i can not accept two answers. But thanks again!
– Lorenz
Apr 14 at 3:07
add a comment |
You can do it this way to add those rows to your original dataframe:
df.set_index(['Country','Industry','Field'])
.unstack()['Value']
.eval('Net = Import - Export')
.stack().rename('Value').reset_index()
Output:
Country Industry Field Value
0 Canada Retail Export 10
1 Canada Retail Import 30
2 Canada Retail Net 20
3 USA Energy Export 5
4 USA Energy Import 20
5 USA Energy Net 15
6 USA Finance Export 50
7 USA Finance Import 100
8 USA Finance Net 50
9 USA Retail Export 10
10 USA Retail Import 80
11 USA Retail Net 70
Thanks - actually, I wanted to append it to the original df. So, nice trick to do this all in one command,
– Lorenz
Apr 14 at 1:41
1
Coldspeed‘s answer was a slight better fit to my overall code. Took from your code how you appended the result to the original df. Very tight result, though. Pitty that i can not accept two answers. But thanks again!
– Lorenz
Apr 14 at 3:07
add a comment |
You can do it this way to add those rows to your original dataframe:
df.set_index(['Country','Industry','Field'])
.unstack()['Value']
.eval('Net = Import - Export')
.stack().rename('Value').reset_index()
Output:
Country Industry Field Value
0 Canada Retail Export 10
1 Canada Retail Import 30
2 Canada Retail Net 20
3 USA Energy Export 5
4 USA Energy Import 20
5 USA Energy Net 15
6 USA Finance Export 50
7 USA Finance Import 100
8 USA Finance Net 50
9 USA Retail Export 10
10 USA Retail Import 80
11 USA Retail Net 70
You can do it this way to add those rows to your original dataframe:
df.set_index(['Country','Industry','Field'])
.unstack()['Value']
.eval('Net = Import - Export')
.stack().rename('Value').reset_index()
Output:
Country Industry Field Value
0 Canada Retail Export 10
1 Canada Retail Import 30
2 Canada Retail Net 20
3 USA Energy Export 5
4 USA Energy Import 20
5 USA Energy Net 15
6 USA Finance Export 50
7 USA Finance Import 100
8 USA Finance Net 50
9 USA Retail Export 10
10 USA Retail Import 80
11 USA Retail Net 70
answered Apr 13 at 22:20


Scott BostonScott Boston
58.9k73258
58.9k73258
Thanks - actually, I wanted to append it to the original df. So, nice trick to do this all in one command,
– Lorenz
Apr 14 at 1:41
1
Coldspeed‘s answer was a slight better fit to my overall code. Took from your code how you appended the result to the original df. Very tight result, though. Pitty that i can not accept two answers. But thanks again!
– Lorenz
Apr 14 at 3:07
add a comment |
Thanks - actually, I wanted to append it to the original df. So, nice trick to do this all in one command,
– Lorenz
Apr 14 at 1:41
1
Coldspeed‘s answer was a slight better fit to my overall code. Took from your code how you appended the result to the original df. Very tight result, though. Pitty that i can not accept two answers. But thanks again!
– Lorenz
Apr 14 at 3:07
Thanks - actually, I wanted to append it to the original df. So, nice trick to do this all in one command,
– Lorenz
Apr 14 at 1:41
Thanks - actually, I wanted to append it to the original df. So, nice trick to do this all in one command,
– Lorenz
Apr 14 at 1:41
1
1
Coldspeed‘s answer was a slight better fit to my overall code. Took from your code how you appended the result to the original df. Very tight result, though. Pitty that i can not accept two answers. But thanks again!
– Lorenz
Apr 14 at 3:07
Coldspeed‘s answer was a slight better fit to my overall code. Took from your code how you appended the result to the original df. Very tight result, though. Pitty that i can not accept two answers. But thanks again!
– Lorenz
Apr 14 at 3:07
add a comment |
This answer takes advantage of the fact that pandas puts the group keys in the multiindex of the resulting dataframe. (If there were only one group key, you could use loc
.)
>>> s = df.groupby(['Country', 'Industry', 'Field'])['Value'].sum()
>>> s.xs('Import', axis=0, level='Field') - s.xs('Export', axis=0, level='Field')
Country Industry
Canada Retail 20
USA Energy 15
Finance 50
Retail 70
Name: Value, dtype: int64
add a comment |
This answer takes advantage of the fact that pandas puts the group keys in the multiindex of the resulting dataframe. (If there were only one group key, you could use loc
.)
>>> s = df.groupby(['Country', 'Industry', 'Field'])['Value'].sum()
>>> s.xs('Import', axis=0, level='Field') - s.xs('Export', axis=0, level='Field')
Country Industry
Canada Retail 20
USA Energy 15
Finance 50
Retail 70
Name: Value, dtype: int64
add a comment |
This answer takes advantage of the fact that pandas puts the group keys in the multiindex of the resulting dataframe. (If there were only one group key, you could use loc
.)
>>> s = df.groupby(['Country', 'Industry', 'Field'])['Value'].sum()
>>> s.xs('Import', axis=0, level='Field') - s.xs('Export', axis=0, level='Field')
Country Industry
Canada Retail 20
USA Energy 15
Finance 50
Retail 70
Name: Value, dtype: int64
This answer takes advantage of the fact that pandas puts the group keys in the multiindex of the resulting dataframe. (If there were only one group key, you could use loc
.)
>>> s = df.groupby(['Country', 'Industry', 'Field'])['Value'].sum()
>>> s.xs('Import', axis=0, level='Field') - s.xs('Export', axis=0, level='Field')
Country Industry
Canada Retail 20
USA Energy 15
Finance 50
Retail 70
Name: Value, dtype: int64
answered Apr 14 at 3:07
BallpointBenBallpointBen
3,7681639
3,7681639
add a comment |
add a comment |
Thanks for contributing an answer to Stack Overflow!
- Please be sure to answer the question. Provide details and share your research!
But avoid …
- Asking for help, clarification, or responding to other answers.
- Making statements based on opinion; back them up with references or personal experience.
To learn more, see our tips on writing great answers.
Sign up or log in
StackExchange.ready(function ()
StackExchange.helpers.onClickDraftSave('#login-link');
);
Sign up using Google
Sign up using Facebook
Sign up using Email and Password
Post as a guest
Required, but never shown
StackExchange.ready(
function ()
StackExchange.openid.initPostLogin('.new-post-login', 'https%3a%2f%2fstackoverflow.com%2fquestions%2f55670192%2fpandas-dataframes-create-new-rows-with-calculations-across-existing-rows%23new-answer', 'question_page');
);
Post as a guest
Required, but never shown
Sign up or log in
StackExchange.ready(function ()
StackExchange.helpers.onClickDraftSave('#login-link');
);
Sign up using Google
Sign up using Facebook
Sign up using Email and Password
Post as a guest
Required, but never shown
Sign up or log in
StackExchange.ready(function ()
StackExchange.helpers.onClickDraftSave('#login-link');
);
Sign up using Google
Sign up using Facebook
Sign up using Email and Password
Post as a guest
Required, but never shown
Sign up or log in
StackExchange.ready(function ()
StackExchange.helpers.onClickDraftSave('#login-link');
);
Sign up using Google
Sign up using Facebook
Sign up using Email and Password
Sign up using Google
Sign up using Facebook
Sign up using Email and Password
Post as a guest
Required, but never shown
Required, but never shown
Required, but never shown
Required, but never shown
Required, but never shown
Required, but never shown
Required, but never shown
Required, but never shown
Required, but never shown
ITuvoA6x