Training a classifier when some of the features are unknown Announcing the arrival of Valued Associate #679: Cesar Manara Planned maintenance scheduled April 23, 2019 at 23:30 UTC (7:30pm US/Eastern) 2019 Moderator Election Q&A - Questionnaire 2019 Community Moderator Election ResultsClassifier ChainsHow to improve an existing (trained) classifier?What is effect when I set up some self defined predisctor variables?Why Matlab neural network classification returns decimal values on prediction dataset?Fitting and transforming text data in training, testing, and validation setsHow to quantify the performance of the classifier (multi-class SVM) using the test data?How do I control for some patients providing multiple samples in my training data?Training and Test setTraining a convolutional neural network for image denoising in MatlabDealing with correlated features when calculating permutation importance
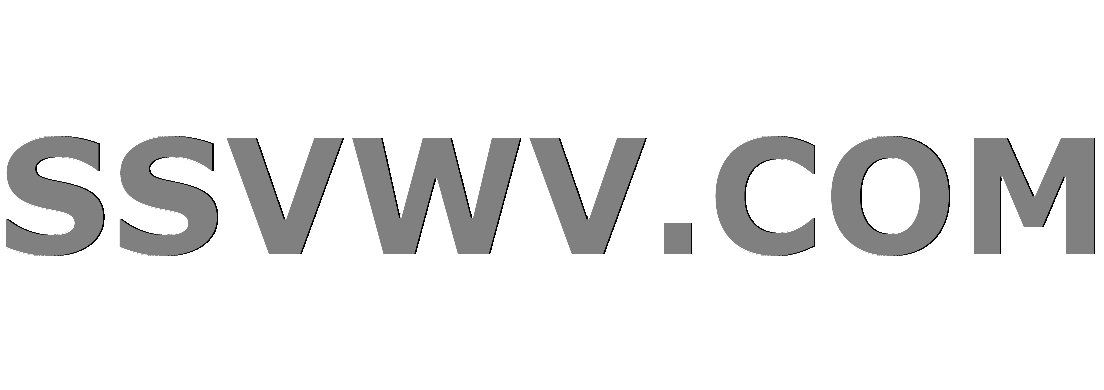
Multi tool use
What is the difference between a "ranged attack" and a "ranged weapon attack"?
Flight departed from the gate 5 min before scheduled departure time. Refund options
How can god fight other gods?
Did Mueller's report provide an evidentiary basis for the claim of Russian govt election interference via social media?
How do living politicians protect their readily obtainable signatures from misuse?
Why is it faster to reheat something than it is to cook it?
Simple HTTP Server
Does the Black Tentacles spell do damage twice at the start of turn to an already restrained creature?
Tips to organize LaTeX presentations for a semester
GDP with Intermediate Production
What does it mean that physics no longer uses mechanical models to describe phenomena?
Printing attributes of selection in ArcPy?
Caught masturbating at work
Mounting TV on a weird wall that has some material between the drywall and stud
Google .dev domain strangely redirects to https
What would you call this weird metallic apparatus that allows you to lift people?
i2c bus hangs in master RPi access to MSP430G uC ~1 in 1000 accesses
Simple Line in LaTeX Help!
Most effective melee weapons for arboreal combat? (pre-gunpowder technology)
what is the log of the PDF for a Normal Distribution?
How can I prevent/balance waiting and turtling as a response to cooldown mechanics
Was Kant an Intuitionist about mathematical objects?
Can two people see the same photon?
Why complex landing gears are used instead of simple,reliability and light weight muscle wire or shape memory alloys?
Training a classifier when some of the features are unknown
Announcing the arrival of Valued Associate #679: Cesar Manara
Planned maintenance scheduled April 23, 2019 at 23:30 UTC (7:30pm US/Eastern)
2019 Moderator Election Q&A - Questionnaire
2019 Community Moderator Election ResultsClassifier ChainsHow to improve an existing (trained) classifier?What is effect when I set up some self defined predisctor variables?Why Matlab neural network classification returns decimal values on prediction dataset?Fitting and transforming text data in training, testing, and validation setsHow to quantify the performance of the classifier (multi-class SVM) using the test data?How do I control for some patients providing multiple samples in my training data?Training and Test setTraining a convolutional neural network for image denoising in MatlabDealing with correlated features when calculating permutation importance
$begingroup$
I am training a classifier in Matlab with a dataset that I created.
Unfortunately some of the features in the dataset were not recorded.
I currently have the unknown features set as -99999.
So, for example my dataset looks something like this:
class1: 10 1 12 -99999 6 8
class1: 11 2 13 7 6 10
...
class2: 5 -99999 4 3 2 -99999
class2: -99999 16 4 3 1 8
...
class3: 18 2 11 22 7 5
class3: 19 1 9 25 7 5
...
and so on, where the -99999 are the places where the features werent able to be measured. In this case, each class has 6 features.
I don't want to bias my classifier with the unknown features so I thought it would be a good idea to set the unknowns to -99999 so it would be way out of the range of normal features.
I tested the classifier with the -99999's and it was 78% accurate.
Then I changed the -99999 to 0's and tested the classifier again, this time it was 91% accurate.
So my question is, what is a general rule for training a classifier when some of the features were not recorded? Was I right to assume setting the unknowns to a very high negative value? But why was it more accurate when I set the unknowns to 0s?
Thanks for reading!
machine-learning classification dataset matlab
New contributor
Darklink9110 is a new contributor to this site. Take care in asking for clarification, commenting, and answering.
Check out our Code of Conduct.
$endgroup$
add a comment |
$begingroup$
I am training a classifier in Matlab with a dataset that I created.
Unfortunately some of the features in the dataset were not recorded.
I currently have the unknown features set as -99999.
So, for example my dataset looks something like this:
class1: 10 1 12 -99999 6 8
class1: 11 2 13 7 6 10
...
class2: 5 -99999 4 3 2 -99999
class2: -99999 16 4 3 1 8
...
class3: 18 2 11 22 7 5
class3: 19 1 9 25 7 5
...
and so on, where the -99999 are the places where the features werent able to be measured. In this case, each class has 6 features.
I don't want to bias my classifier with the unknown features so I thought it would be a good idea to set the unknowns to -99999 so it would be way out of the range of normal features.
I tested the classifier with the -99999's and it was 78% accurate.
Then I changed the -99999 to 0's and tested the classifier again, this time it was 91% accurate.
So my question is, what is a general rule for training a classifier when some of the features were not recorded? Was I right to assume setting the unknowns to a very high negative value? But why was it more accurate when I set the unknowns to 0s?
Thanks for reading!
machine-learning classification dataset matlab
New contributor
Darklink9110 is a new contributor to this site. Take care in asking for clarification, commenting, and answering.
Check out our Code of Conduct.
$endgroup$
add a comment |
$begingroup$
I am training a classifier in Matlab with a dataset that I created.
Unfortunately some of the features in the dataset were not recorded.
I currently have the unknown features set as -99999.
So, for example my dataset looks something like this:
class1: 10 1 12 -99999 6 8
class1: 11 2 13 7 6 10
...
class2: 5 -99999 4 3 2 -99999
class2: -99999 16 4 3 1 8
...
class3: 18 2 11 22 7 5
class3: 19 1 9 25 7 5
...
and so on, where the -99999 are the places where the features werent able to be measured. In this case, each class has 6 features.
I don't want to bias my classifier with the unknown features so I thought it would be a good idea to set the unknowns to -99999 so it would be way out of the range of normal features.
I tested the classifier with the -99999's and it was 78% accurate.
Then I changed the -99999 to 0's and tested the classifier again, this time it was 91% accurate.
So my question is, what is a general rule for training a classifier when some of the features were not recorded? Was I right to assume setting the unknowns to a very high negative value? But why was it more accurate when I set the unknowns to 0s?
Thanks for reading!
machine-learning classification dataset matlab
New contributor
Darklink9110 is a new contributor to this site. Take care in asking for clarification, commenting, and answering.
Check out our Code of Conduct.
$endgroup$
I am training a classifier in Matlab with a dataset that I created.
Unfortunately some of the features in the dataset were not recorded.
I currently have the unknown features set as -99999.
So, for example my dataset looks something like this:
class1: 10 1 12 -99999 6 8
class1: 11 2 13 7 6 10
...
class2: 5 -99999 4 3 2 -99999
class2: -99999 16 4 3 1 8
...
class3: 18 2 11 22 7 5
class3: 19 1 9 25 7 5
...
and so on, where the -99999 are the places where the features werent able to be measured. In this case, each class has 6 features.
I don't want to bias my classifier with the unknown features so I thought it would be a good idea to set the unknowns to -99999 so it would be way out of the range of normal features.
I tested the classifier with the -99999's and it was 78% accurate.
Then I changed the -99999 to 0's and tested the classifier again, this time it was 91% accurate.
So my question is, what is a general rule for training a classifier when some of the features were not recorded? Was I right to assume setting the unknowns to a very high negative value? But why was it more accurate when I set the unknowns to 0s?
Thanks for reading!
machine-learning classification dataset matlab
machine-learning classification dataset matlab
New contributor
Darklink9110 is a new contributor to this site. Take care in asking for clarification, commenting, and answering.
Check out our Code of Conduct.
New contributor
Darklink9110 is a new contributor to this site. Take care in asking for clarification, commenting, and answering.
Check out our Code of Conduct.
edited Apr 15 at 2:46
Darklink9110
New contributor
Darklink9110 is a new contributor to this site. Take care in asking for clarification, commenting, and answering.
Check out our Code of Conduct.
asked Apr 15 at 2:40
Darklink9110Darklink9110
235
235
New contributor
Darklink9110 is a new contributor to this site. Take care in asking for clarification, commenting, and answering.
Check out our Code of Conduct.
New contributor
Darklink9110 is a new contributor to this site. Take care in asking for clarification, commenting, and answering.
Check out our Code of Conduct.
Darklink9110 is a new contributor to this site. Take care in asking for clarification, commenting, and answering.
Check out our Code of Conduct.
add a comment |
add a comment |
1 Answer
1
active
oldest
votes
$begingroup$
Welcome to Data Science SE!
Well, we say that most of our jobs is to wrangle with data, and that is because data is usually trying to deceive us... jokes aside:
You have a missing data problem
that means your have to clean your data and fill those missing values. To perform this cleaning process your must take the most classic statistician inside of you and ask:
- Why is this data missing?
- How much data is missing?
There are many reasons for a specific information to be unavailable. This will demand you to make assumptions and decide how to deal with this.
Jeff Sauro posted at MeasuringU: 7 Ways to Handle Missing Data, some which I list here:
- Delete corrupted samples:
If you have a large dataset and there is not much data missing, you can simply remove those corrupted data points and go on with life
- Recover the values:
Some problems will allow you to go back and get missing information.
We usually ain't that lucky, then you can
- Educated Guessing:
Sometimes, you can infer what would be the feature value by simply looking their pears. That is a bit arbitrary but it might work.
- Average:
This is the most common approach, simply use the average of that value whenever it is missing. This might artificially reduce your variance but so does using 0 or -9999... for every missing value.
- Regression Substitution:
You can use a multiple regression to infer the missing value from the available values for each candidate.
Some references on missing data are:
- Allison, Paul D. 2001. Missing Data. Sage University Papers
Series on Quantitative Applications in the Social Sciences.
Thousand Oaks: Sage. - Enders, Craig. 2010. Applied Missing Data Analysis.
Guilford Press: New York. - Little, Roderick J., Donald Rubin. 2002. Statistical Analysis
with Missing Data. John Wiley & Sons, Inc: Hoboken. - Schafer, Joseph L., John W. Graham. 2002. “Missing Data:
Our View of the State of the Art.” Psychological Methods.
About your experiment:
Adding -99... is creating outliers and that bit of information is heavy (numerically speaking, it is huge) and will affect parameter tuning. For example, suppose you have this data:
| Feature1 | Feature2 |
|----------|----------|
| 0 | 8 |
| -1 | 7 |
| 1 | - |
| - | 8 |
And you try filling the missing values with -99, now try to fit a linear regression trough the data. Can you see that you don't be able to fit it properly?
The line won't fit, and this will yield bad performance.
Adding 0 values on the other hand will give a slightly better line:
It is still not good, but slightly better since the scale of the parameters will be more realistic.
Now, using average, is this case will give you even better curve, but using regression will give you a perfect fitting line:
Note: I need to remake those images, but these should do until I have the time for it.
$endgroup$
2
$begingroup$
Excellent explanation. Also, just adding to it, I think the best thing to do in data science is to play with missing data. You can (and should) check out Multiple Imputation, this is the state of the art process to deal with missing data.
$endgroup$
– jeff
Apr 15 at 7:20
add a comment |
Your Answer
StackExchange.ready(function()
var channelOptions =
tags: "".split(" "),
id: "557"
;
initTagRenderer("".split(" "), "".split(" "), channelOptions);
StackExchange.using("externalEditor", function()
// Have to fire editor after snippets, if snippets enabled
if (StackExchange.settings.snippets.snippetsEnabled)
StackExchange.using("snippets", function()
createEditor();
);
else
createEditor();
);
function createEditor()
StackExchange.prepareEditor(
heartbeatType: 'answer',
autoActivateHeartbeat: false,
convertImagesToLinks: false,
noModals: true,
showLowRepImageUploadWarning: true,
reputationToPostImages: null,
bindNavPrevention: true,
postfix: "",
imageUploader:
brandingHtml: "Powered by u003ca class="icon-imgur-white" href="https://imgur.com/"u003eu003c/au003e",
contentPolicyHtml: "User contributions licensed under u003ca href="https://creativecommons.org/licenses/by-sa/3.0/"u003ecc by-sa 3.0 with attribution requiredu003c/au003e u003ca href="https://stackoverflow.com/legal/content-policy"u003e(content policy)u003c/au003e",
allowUrls: true
,
onDemand: true,
discardSelector: ".discard-answer"
,immediatelyShowMarkdownHelp:true
);
);
Darklink9110 is a new contributor. Be nice, and check out our Code of Conduct.
Sign up or log in
StackExchange.ready(function ()
StackExchange.helpers.onClickDraftSave('#login-link');
);
Sign up using Google
Sign up using Facebook
Sign up using Email and Password
Post as a guest
Required, but never shown
StackExchange.ready(
function ()
StackExchange.openid.initPostLogin('.new-post-login', 'https%3a%2f%2fdatascience.stackexchange.com%2fquestions%2f49298%2ftraining-a-classifier-when-some-of-the-features-are-unknown%23new-answer', 'question_page');
);
Post as a guest
Required, but never shown
1 Answer
1
active
oldest
votes
1 Answer
1
active
oldest
votes
active
oldest
votes
active
oldest
votes
$begingroup$
Welcome to Data Science SE!
Well, we say that most of our jobs is to wrangle with data, and that is because data is usually trying to deceive us... jokes aside:
You have a missing data problem
that means your have to clean your data and fill those missing values. To perform this cleaning process your must take the most classic statistician inside of you and ask:
- Why is this data missing?
- How much data is missing?
There are many reasons for a specific information to be unavailable. This will demand you to make assumptions and decide how to deal with this.
Jeff Sauro posted at MeasuringU: 7 Ways to Handle Missing Data, some which I list here:
- Delete corrupted samples:
If you have a large dataset and there is not much data missing, you can simply remove those corrupted data points and go on with life
- Recover the values:
Some problems will allow you to go back and get missing information.
We usually ain't that lucky, then you can
- Educated Guessing:
Sometimes, you can infer what would be the feature value by simply looking their pears. That is a bit arbitrary but it might work.
- Average:
This is the most common approach, simply use the average of that value whenever it is missing. This might artificially reduce your variance but so does using 0 or -9999... for every missing value.
- Regression Substitution:
You can use a multiple regression to infer the missing value from the available values for each candidate.
Some references on missing data are:
- Allison, Paul D. 2001. Missing Data. Sage University Papers
Series on Quantitative Applications in the Social Sciences.
Thousand Oaks: Sage. - Enders, Craig. 2010. Applied Missing Data Analysis.
Guilford Press: New York. - Little, Roderick J., Donald Rubin. 2002. Statistical Analysis
with Missing Data. John Wiley & Sons, Inc: Hoboken. - Schafer, Joseph L., John W. Graham. 2002. “Missing Data:
Our View of the State of the Art.” Psychological Methods.
About your experiment:
Adding -99... is creating outliers and that bit of information is heavy (numerically speaking, it is huge) and will affect parameter tuning. For example, suppose you have this data:
| Feature1 | Feature2 |
|----------|----------|
| 0 | 8 |
| -1 | 7 |
| 1 | - |
| - | 8 |
And you try filling the missing values with -99, now try to fit a linear regression trough the data. Can you see that you don't be able to fit it properly?
The line won't fit, and this will yield bad performance.
Adding 0 values on the other hand will give a slightly better line:
It is still not good, but slightly better since the scale of the parameters will be more realistic.
Now, using average, is this case will give you even better curve, but using regression will give you a perfect fitting line:
Note: I need to remake those images, but these should do until I have the time for it.
$endgroup$
2
$begingroup$
Excellent explanation. Also, just adding to it, I think the best thing to do in data science is to play with missing data. You can (and should) check out Multiple Imputation, this is the state of the art process to deal with missing data.
$endgroup$
– jeff
Apr 15 at 7:20
add a comment |
$begingroup$
Welcome to Data Science SE!
Well, we say that most of our jobs is to wrangle with data, and that is because data is usually trying to deceive us... jokes aside:
You have a missing data problem
that means your have to clean your data and fill those missing values. To perform this cleaning process your must take the most classic statistician inside of you and ask:
- Why is this data missing?
- How much data is missing?
There are many reasons for a specific information to be unavailable. This will demand you to make assumptions and decide how to deal with this.
Jeff Sauro posted at MeasuringU: 7 Ways to Handle Missing Data, some which I list here:
- Delete corrupted samples:
If you have a large dataset and there is not much data missing, you can simply remove those corrupted data points and go on with life
- Recover the values:
Some problems will allow you to go back and get missing information.
We usually ain't that lucky, then you can
- Educated Guessing:
Sometimes, you can infer what would be the feature value by simply looking their pears. That is a bit arbitrary but it might work.
- Average:
This is the most common approach, simply use the average of that value whenever it is missing. This might artificially reduce your variance but so does using 0 or -9999... for every missing value.
- Regression Substitution:
You can use a multiple regression to infer the missing value from the available values for each candidate.
Some references on missing data are:
- Allison, Paul D. 2001. Missing Data. Sage University Papers
Series on Quantitative Applications in the Social Sciences.
Thousand Oaks: Sage. - Enders, Craig. 2010. Applied Missing Data Analysis.
Guilford Press: New York. - Little, Roderick J., Donald Rubin. 2002. Statistical Analysis
with Missing Data. John Wiley & Sons, Inc: Hoboken. - Schafer, Joseph L., John W. Graham. 2002. “Missing Data:
Our View of the State of the Art.” Psychological Methods.
About your experiment:
Adding -99... is creating outliers and that bit of information is heavy (numerically speaking, it is huge) and will affect parameter tuning. For example, suppose you have this data:
| Feature1 | Feature2 |
|----------|----------|
| 0 | 8 |
| -1 | 7 |
| 1 | - |
| - | 8 |
And you try filling the missing values with -99, now try to fit a linear regression trough the data. Can you see that you don't be able to fit it properly?
The line won't fit, and this will yield bad performance.
Adding 0 values on the other hand will give a slightly better line:
It is still not good, but slightly better since the scale of the parameters will be more realistic.
Now, using average, is this case will give you even better curve, but using regression will give you a perfect fitting line:
Note: I need to remake those images, but these should do until I have the time for it.
$endgroup$
2
$begingroup$
Excellent explanation. Also, just adding to it, I think the best thing to do in data science is to play with missing data. You can (and should) check out Multiple Imputation, this is the state of the art process to deal with missing data.
$endgroup$
– jeff
Apr 15 at 7:20
add a comment |
$begingroup$
Welcome to Data Science SE!
Well, we say that most of our jobs is to wrangle with data, and that is because data is usually trying to deceive us... jokes aside:
You have a missing data problem
that means your have to clean your data and fill those missing values. To perform this cleaning process your must take the most classic statistician inside of you and ask:
- Why is this data missing?
- How much data is missing?
There are many reasons for a specific information to be unavailable. This will demand you to make assumptions and decide how to deal with this.
Jeff Sauro posted at MeasuringU: 7 Ways to Handle Missing Data, some which I list here:
- Delete corrupted samples:
If you have a large dataset and there is not much data missing, you can simply remove those corrupted data points and go on with life
- Recover the values:
Some problems will allow you to go back and get missing information.
We usually ain't that lucky, then you can
- Educated Guessing:
Sometimes, you can infer what would be the feature value by simply looking their pears. That is a bit arbitrary but it might work.
- Average:
This is the most common approach, simply use the average of that value whenever it is missing. This might artificially reduce your variance but so does using 0 or -9999... for every missing value.
- Regression Substitution:
You can use a multiple regression to infer the missing value from the available values for each candidate.
Some references on missing data are:
- Allison, Paul D. 2001. Missing Data. Sage University Papers
Series on Quantitative Applications in the Social Sciences.
Thousand Oaks: Sage. - Enders, Craig. 2010. Applied Missing Data Analysis.
Guilford Press: New York. - Little, Roderick J., Donald Rubin. 2002. Statistical Analysis
with Missing Data. John Wiley & Sons, Inc: Hoboken. - Schafer, Joseph L., John W. Graham. 2002. “Missing Data:
Our View of the State of the Art.” Psychological Methods.
About your experiment:
Adding -99... is creating outliers and that bit of information is heavy (numerically speaking, it is huge) and will affect parameter tuning. For example, suppose you have this data:
| Feature1 | Feature2 |
|----------|----------|
| 0 | 8 |
| -1 | 7 |
| 1 | - |
| - | 8 |
And you try filling the missing values with -99, now try to fit a linear regression trough the data. Can you see that you don't be able to fit it properly?
The line won't fit, and this will yield bad performance.
Adding 0 values on the other hand will give a slightly better line:
It is still not good, but slightly better since the scale of the parameters will be more realistic.
Now, using average, is this case will give you even better curve, but using regression will give you a perfect fitting line:
Note: I need to remake those images, but these should do until I have the time for it.
$endgroup$
Welcome to Data Science SE!
Well, we say that most of our jobs is to wrangle with data, and that is because data is usually trying to deceive us... jokes aside:
You have a missing data problem
that means your have to clean your data and fill those missing values. To perform this cleaning process your must take the most classic statistician inside of you and ask:
- Why is this data missing?
- How much data is missing?
There are many reasons for a specific information to be unavailable. This will demand you to make assumptions and decide how to deal with this.
Jeff Sauro posted at MeasuringU: 7 Ways to Handle Missing Data, some which I list here:
- Delete corrupted samples:
If you have a large dataset and there is not much data missing, you can simply remove those corrupted data points and go on with life
- Recover the values:
Some problems will allow you to go back and get missing information.
We usually ain't that lucky, then you can
- Educated Guessing:
Sometimes, you can infer what would be the feature value by simply looking their pears. That is a bit arbitrary but it might work.
- Average:
This is the most common approach, simply use the average of that value whenever it is missing. This might artificially reduce your variance but so does using 0 or -9999... for every missing value.
- Regression Substitution:
You can use a multiple regression to infer the missing value from the available values for each candidate.
Some references on missing data are:
- Allison, Paul D. 2001. Missing Data. Sage University Papers
Series on Quantitative Applications in the Social Sciences.
Thousand Oaks: Sage. - Enders, Craig. 2010. Applied Missing Data Analysis.
Guilford Press: New York. - Little, Roderick J., Donald Rubin. 2002. Statistical Analysis
with Missing Data. John Wiley & Sons, Inc: Hoboken. - Schafer, Joseph L., John W. Graham. 2002. “Missing Data:
Our View of the State of the Art.” Psychological Methods.
About your experiment:
Adding -99... is creating outliers and that bit of information is heavy (numerically speaking, it is huge) and will affect parameter tuning. For example, suppose you have this data:
| Feature1 | Feature2 |
|----------|----------|
| 0 | 8 |
| -1 | 7 |
| 1 | - |
| - | 8 |
And you try filling the missing values with -99, now try to fit a linear regression trough the data. Can you see that you don't be able to fit it properly?
The line won't fit, and this will yield bad performance.
Adding 0 values on the other hand will give a slightly better line:
It is still not good, but slightly better since the scale of the parameters will be more realistic.
Now, using average, is this case will give you even better curve, but using regression will give you a perfect fitting line:
Note: I need to remake those images, but these should do until I have the time for it.
edited Apr 15 at 3:56
answered Apr 15 at 3:30
Pedro Henrique MonfortePedro Henrique Monforte
559118
559118
2
$begingroup$
Excellent explanation. Also, just adding to it, I think the best thing to do in data science is to play with missing data. You can (and should) check out Multiple Imputation, this is the state of the art process to deal with missing data.
$endgroup$
– jeff
Apr 15 at 7:20
add a comment |
2
$begingroup$
Excellent explanation. Also, just adding to it, I think the best thing to do in data science is to play with missing data. You can (and should) check out Multiple Imputation, this is the state of the art process to deal with missing data.
$endgroup$
– jeff
Apr 15 at 7:20
2
2
$begingroup$
Excellent explanation. Also, just adding to it, I think the best thing to do in data science is to play with missing data. You can (and should) check out Multiple Imputation, this is the state of the art process to deal with missing data.
$endgroup$
– jeff
Apr 15 at 7:20
$begingroup$
Excellent explanation. Also, just adding to it, I think the best thing to do in data science is to play with missing data. You can (and should) check out Multiple Imputation, this is the state of the art process to deal with missing data.
$endgroup$
– jeff
Apr 15 at 7:20
add a comment |
Darklink9110 is a new contributor. Be nice, and check out our Code of Conduct.
Darklink9110 is a new contributor. Be nice, and check out our Code of Conduct.
Darklink9110 is a new contributor. Be nice, and check out our Code of Conduct.
Darklink9110 is a new contributor. Be nice, and check out our Code of Conduct.
Thanks for contributing an answer to Data Science Stack Exchange!
- Please be sure to answer the question. Provide details and share your research!
But avoid …
- Asking for help, clarification, or responding to other answers.
- Making statements based on opinion; back them up with references or personal experience.
Use MathJax to format equations. MathJax reference.
To learn more, see our tips on writing great answers.
Sign up or log in
StackExchange.ready(function ()
StackExchange.helpers.onClickDraftSave('#login-link');
);
Sign up using Google
Sign up using Facebook
Sign up using Email and Password
Post as a guest
Required, but never shown
StackExchange.ready(
function ()
StackExchange.openid.initPostLogin('.new-post-login', 'https%3a%2f%2fdatascience.stackexchange.com%2fquestions%2f49298%2ftraining-a-classifier-when-some-of-the-features-are-unknown%23new-answer', 'question_page');
);
Post as a guest
Required, but never shown
Sign up or log in
StackExchange.ready(function ()
StackExchange.helpers.onClickDraftSave('#login-link');
);
Sign up using Google
Sign up using Facebook
Sign up using Email and Password
Post as a guest
Required, but never shown
Sign up or log in
StackExchange.ready(function ()
StackExchange.helpers.onClickDraftSave('#login-link');
);
Sign up using Google
Sign up using Facebook
Sign up using Email and Password
Post as a guest
Required, but never shown
Sign up or log in
StackExchange.ready(function ()
StackExchange.helpers.onClickDraftSave('#login-link');
);
Sign up using Google
Sign up using Facebook
Sign up using Email and Password
Sign up using Google
Sign up using Facebook
Sign up using Email and Password
Post as a guest
Required, but never shown
Required, but never shown
Required, but never shown
Required, but never shown
Required, but never shown
Required, but never shown
Required, but never shown
Required, but never shown
Required, but never shown
z7,pt,tNU,Xh8yKYw8BV